A method for semantic segmentation of scene point cloud
A semantic segmentation and scene point technology, applied in the field of computer vision, can solve the problems of understanding data resolution limitations, difficult to deal with large-scale dense point clouds, and local features are not robust enough to achieve remarkable results.
- Summary
- Abstract
- Description
- Claims
- Application Information
AI Technical Summary
Problems solved by technology
Method used
Image
Examples
Embodiment Construction
[0056] The invention will be described in further detail below in conjunction with specific embodiments, but the present invention is not limited to specific embodiments.
[0057] A method for semantic segmentation of large-scale dense scene point clouds based on deep learning, including the training of the network model and the operation steps of the model.
[0058] 1. Training network model
[0059] To train the semantic segmentation network for this large-scale dense scene point cloud, it is first necessary to prepare sufficient point cloud data. Each scene point cloud sample should contain RGBXYZ and semantic category information to which each point belongs. Taking the S3DIS indoor scene dataset as an example, after data enhancement, a total of 2654 scene point cloud samples are used as the training set, and 578 samples are used as the verification set.
[0060] After obtaining enough data sets, it is first necessary to convert the features of each point into RGBDHN info...
PUM
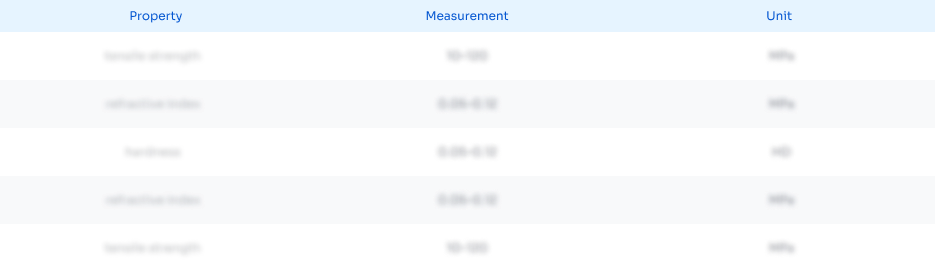
Abstract
Description
Claims
Application Information
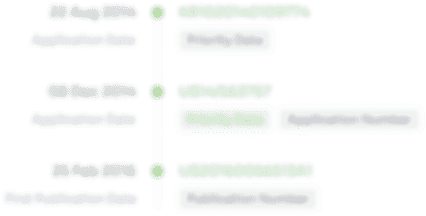
- R&D Engineer
- R&D Manager
- IP Professional
- Industry Leading Data Capabilities
- Powerful AI technology
- Patent DNA Extraction
Browse by: Latest US Patents, China's latest patents, Technical Efficacy Thesaurus, Application Domain, Technology Topic, Popular Technical Reports.
© 2024 PatSnap. All rights reserved.Legal|Privacy policy|Modern Slavery Act Transparency Statement|Sitemap|About US| Contact US: help@patsnap.com