Depth convolution network model of multi-motion streams for video prediction
A deep convolution and network model technology, applied in the fields of artificial intelligence technology and video analysis, can solve problems such as difficulty in achieving clear and accurate long-term predictions, fuzzy prediction results, fuzzy prediction images, etc., to achieve clear prediction results and improve robustness. Sticky, extended frame rate effect
- Summary
- Abstract
- Description
- Claims
- Application Information
AI Technical Summary
Problems solved by technology
Method used
Image
Examples
Embodiment Construction
[0036] Below in conjunction with accompanying drawing, further describe the present invention through embodiment, but do not limit the scope of the present invention in any way.
[0037] The present invention proposes a multi-motion flow deep convolutional network model method (MMF for short) for video prediction, which is mainly used to realize the prediction of several frames of video in the future by several frames of video sequences; figure 1 and figure 2 They are respectively the network structure diagram of the multi-motion stream video prediction deep convolutional network model provided by the present invention and the processing flow diagram of the multi-motion stream mechanism and base image method. It mainly includes the following steps:
[0038] 1) Using a convolutional auto-encoding network, several input frames are sequentially input into the encoder for encoding, and the feature map is extracted, and the feature map of the previous frame is input into the LSTM...
PUM
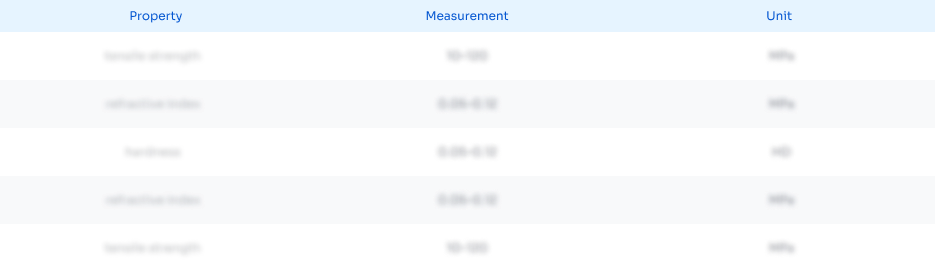
Abstract
Description
Claims
Application Information
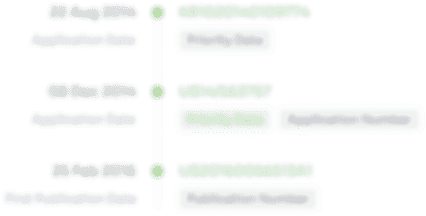
- R&D
- Intellectual Property
- Life Sciences
- Materials
- Tech Scout
- Unparalleled Data Quality
- Higher Quality Content
- 60% Fewer Hallucinations
Browse by: Latest US Patents, China's latest patents, Technical Efficacy Thesaurus, Application Domain, Technology Topic, Popular Technical Reports.
© 2025 PatSnap. All rights reserved.Legal|Privacy policy|Modern Slavery Act Transparency Statement|Sitemap|About US| Contact US: help@patsnap.com