Conditional generative adversarial network-based monocular image depth estimation method
A conditional generation, image depth technology, applied in image enhancement, image analysis, image data processing and other directions, can solve the problem of time-consuming, lack of generality, etc., to achieve the effect of improving quality
- Summary
- Abstract
- Description
- Claims
- Application Information
AI Technical Summary
Problems solved by technology
Method used
Image
Examples
Embodiment Construction
[0024] In order to make the purpose, technical solutions and advantages of the embodiments of the present invention more clear, the specific implementation manners of the present invention will be further described below in conjunction with the embodiments and accompanying drawings.
[0025] Monocular image depth estimation is an ill-posed problem, and countless depth images can be obtained from a single color image. In recent years, a common practice is to use a deep convolutional neural network to directly regress with the real depth image in a certain distance space, but the final result obtained by this method is the average of all possible depth information, so the image is usually blurred. The present invention utilizes a generated confrontation network and a discriminator to judge whether the generated depth map is a scene image corresponding to the original color image, which can better solve the shortcomings of the existing methods.
[0026] The specific technical det...
PUM
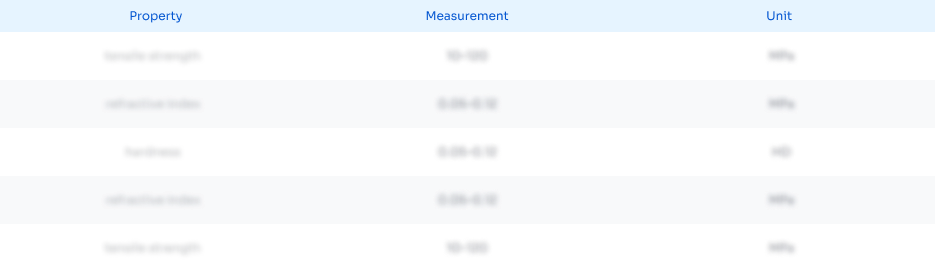
Abstract
Description
Claims
Application Information
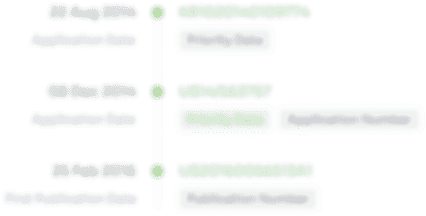
- R&D
- Intellectual Property
- Life Sciences
- Materials
- Tech Scout
- Unparalleled Data Quality
- Higher Quality Content
- 60% Fewer Hallucinations
Browse by: Latest US Patents, China's latest patents, Technical Efficacy Thesaurus, Application Domain, Technology Topic, Popular Technical Reports.
© 2025 PatSnap. All rights reserved.Legal|Privacy policy|Modern Slavery Act Transparency Statement|Sitemap|About US| Contact US: help@patsnap.com