Latent structure mapping algorithm-based soft measurement method for DXN discharge concentration in municipal waste solid incineration process
A technology of emission concentration and soft measurement, applied in chemical process analysis/design, calculation, special data processing applications, etc., can solve problems such as generalization performance needs to be improved, difficult to effectively select, limited universality, etc.
- Summary
- Abstract
- Description
- Claims
- Application Information
AI Technical Summary
Problems solved by technology
Method used
Image
Examples
Embodiment Construction
[0012] Solid waste incineration process and DXN emission description
[0013] The MWSI process includes solid waste storage and transportation, furnace incineration, steam power generation, flue gas treatment and other stages, among which: the incinerator is the core equipment of MWSI, which converts combustible solid waste into ash, flue gas and heat; the grate at the bottom of the incinerator The solid waste is moved in the combustion chamber and burned more effectively and fully; the steam generated by the waste heat boiler is used to generate electricity; some pollutants in the flue gas are removed before being discharged into the atmosphere.
[0014] The MSWI process description for DXN emission concentration soft-sensing is described here. Overall, DXN emission concentrations are related to process variables in the furnace combustion and flue gas treatment stages, as well as to some easily detectable gases emitted from the stack, such as figure 1 shown.
[0015] figur...
PUM
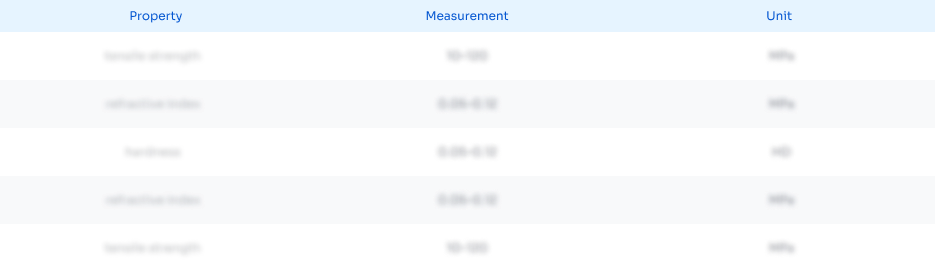
Abstract
Description
Claims
Application Information
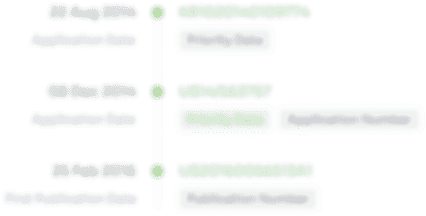
- R&D Engineer
- R&D Manager
- IP Professional
- Industry Leading Data Capabilities
- Powerful AI technology
- Patent DNA Extraction
Browse by: Latest US Patents, China's latest patents, Technical Efficacy Thesaurus, Application Domain, Technology Topic, Popular Technical Reports.
© 2024 PatSnap. All rights reserved.Legal|Privacy policy|Modern Slavery Act Transparency Statement|Sitemap|About US| Contact US: help@patsnap.com