Life prediction method of accelerated life test based on grey RBF neural network
An accelerated life test and neural network technology, applied in the field of accelerated life test evaluation, can solve problems such as difficulty in establishing accelerated models and solving multivariate likelihood equations, large prediction errors, loss of empirical data, etc., so as to facilitate practical engineering applications and avoid Effects of systematic error and life prediction accuracy improvement
- Summary
- Abstract
- Description
- Claims
- Application Information
AI Technical Summary
Problems solved by technology
Method used
Image
Examples
Embodiment 1
[0081] The method in this embodiment uses a Monte Carlo simulation method to verify its correctness. Assume that the lifetime of a product follows a two-parameter Weibull distribution:
[0082] F(t)=1-exp{(t / η) m}, t≥0
[0083] Among them, m is the shape parameter, because it is assumed that the failure mechanism of the product remains unchanged in the accelerated life test, so m does not change with the change of stress and is a constant. η is the characteristic life, this paper considers that the product is only affected by temperature and one kind of stress, and it satisfies the Arronis theorem:
[0084] η=A·exp(E / k·T)
[0085] Where A is a constant; E is activation energy, unit eV; k is Boltzmann's constant, k=8.6171×10 -5 eV / K; T is the thermodynamic temperature in K. Then, the simulation model is:
[0086] F(t)=1-exp{[t / A·exp(E / k·T)] m}, t≥0
[0087] The parameter values are shown in Table 1:
[0088] Table 1: Simulation parameter values
[0089] par...
PUM
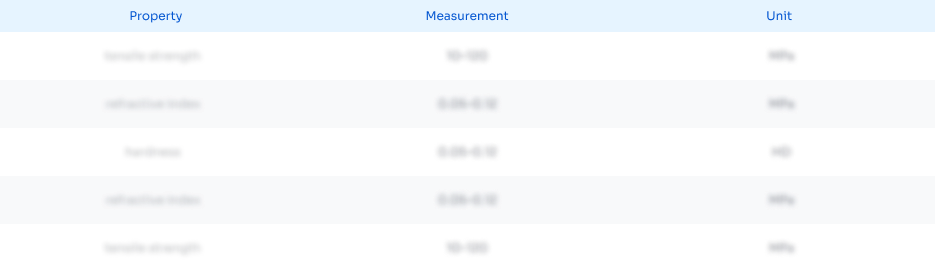
Abstract
Description
Claims
Application Information
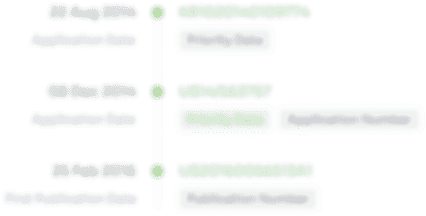
- R&D Engineer
- R&D Manager
- IP Professional
- Industry Leading Data Capabilities
- Powerful AI technology
- Patent DNA Extraction
Browse by: Latest US Patents, China's latest patents, Technical Efficacy Thesaurus, Application Domain, Technology Topic, Popular Technical Reports.
© 2024 PatSnap. All rights reserved.Legal|Privacy policy|Modern Slavery Act Transparency Statement|Sitemap|About US| Contact US: help@patsnap.com