EEG classification method based on Fisher discrimination sparse extreme learning machine
A technology of extreme learning machine and classification method, which is applied in the field of EEG classification based on Fisher discriminant sparse EEG signal pattern recognition, which can solve the problems of insufficient learning and improve classification accuracy, Broad application prospects and the effect of improving accuracy
- Summary
- Abstract
- Description
- Claims
- Application Information
AI Technical Summary
Problems solved by technology
Method used
Image
Examples
Embodiment Construction
[0033]The motor imagery EEG classification method based on the Fisher discriminant sparse extreme learning machine of the present invention will be described in detail below in conjunction with the accompanying drawings. figure 1 for the implementation flow chart.
[0034] Such as figure 1 , the implementation of the inventive method mainly comprises four steps: (1) adopt Fisher discriminant dictionary learning algorithm training structured dictionary; (2) reconstruct signal, draw new characteristic signal; (3) adopt extreme learning machine algorithm to obtain Output the weight matrix of the output layer; (4) Use the trained classification model to distinguish the class label of the test sample.
[0035] Each step will be described in detail below one by one.
[0036] Step 1: Train the structured dictionary using Fisher's discriminant dictionary learning algorithm;
[0037] Specifically: given {A,Y} as a training sample, where A=[A 1 ,A 2 ,...,A c ],A i Represents the ...
PUM
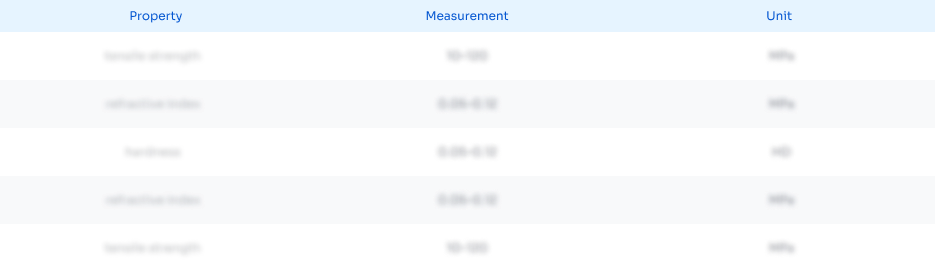
Abstract
Description
Claims
Application Information
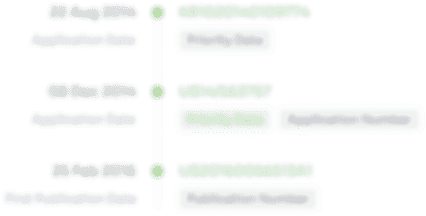
- R&D Engineer
- R&D Manager
- IP Professional
- Industry Leading Data Capabilities
- Powerful AI technology
- Patent DNA Extraction
Browse by: Latest US Patents, China's latest patents, Technical Efficacy Thesaurus, Application Domain, Technology Topic, Popular Technical Reports.
© 2024 PatSnap. All rights reserved.Legal|Privacy policy|Modern Slavery Act Transparency Statement|Sitemap|About US| Contact US: help@patsnap.com