Feature Learning Method for Large-Scale Hybrid Graphs Based on Structural Semantic Fusion
A technology of structural semantics and feature learning, applied in character and pattern recognition, instrumentation, computing, etc., can solve problems such as low quality of graph feature learning and inability to mine graph node label information.
- Summary
- Abstract
- Description
- Claims
- Application Information
AI Technical Summary
Problems solved by technology
Method used
Image
Examples
Embodiment 1
[0057] figure 1 It is a schematic diagram of a large-scale hybrid graph feature learning method based on structural semantic fusion according to an embodiment of the present invention, as shown in figure 1 As shown, the embodiment of the present invention provides a large-scale hybrid graph feature learning method of structural semantic fusion, including:
[0058] Step S10, obtaining training semantic label information set S train , the S train is the training node set V train A set of corresponding semantic label information, the V train It is a collection of several nodes randomly sampled from the graph according to the preset sampling ratio. The graph is G, G=(V, E, S), where V is a graph node, E is an edge in the graph, and S is the graph semantic label information;
[0059] Step S20, obtain node pair set P e , P e ={(u,v)}, wherein the node pair (u,v) is the node pair set P e An element in , node u and node v are two nodes corresponding to a certain edge obtained ...
Embodiment 2
[0123] image 3 It is a schematic diagram of a large-scale hybrid graph feature learning device based on structural semantic fusion according to an embodiment of the present invention; as image 3 As shown, the embodiment of the present invention provides a large-scale mixed graph feature learning device based on structural semantic fusion, which is used to complete the method described in the above embodiment, including an acquisition module 10, a traversal module 20, a calculation module 30 and an update module 40 ,in,
[0124] The acquisition module 10 is used to acquire the training semantic label information set S train , the S train is the training node set V train A set of corresponding semantic label information, the V train It is a collection of several nodes randomly sampled from the graph according to the preset sampling ratio. The graph is G, G=(V, E, S), where V is a graph node, E is an edge in the graph, and S is the graph semantic label information;
[012...
Embodiment 3
[0134] Figure 4 A schematic structural diagram of an electronic device for large-scale hybrid graph feature learning based on structural semantic fusion provided by an embodiment of the present invention, as shown in Figure 4 As shown, the device includes: a processor (processor) 801, a memory (memory) 802 and a bus 803;
[0135] Wherein, the processor 801 and the memory 802 complete mutual communication through the bus 803;
[0136] The processor 801 is used to call the program instructions in the memory 802 to execute the methods provided by the above method embodiments, including, for example:
[0137] Obtain training semantic label information set S train , the S train is the training node set V train A set of corresponding semantic label information, the V train It is a collection of several nodes randomly sampled from the graph according to the preset sampling ratio. The graph is G, G=(V, E, S), where V is a graph node, E is an edge in the graph, and S is the gra...
PUM
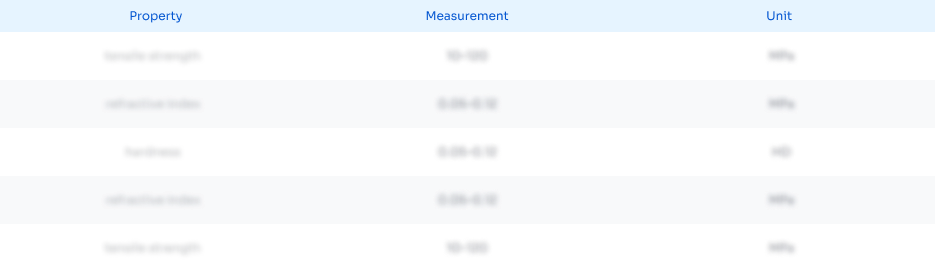
Abstract
Description
Claims
Application Information
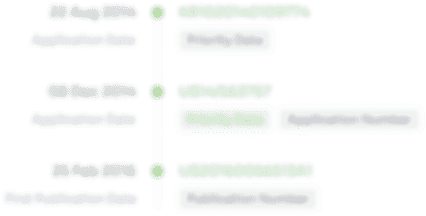
- R&D Engineer
- R&D Manager
- IP Professional
- Industry Leading Data Capabilities
- Powerful AI technology
- Patent DNA Extraction
Browse by: Latest US Patents, China's latest patents, Technical Efficacy Thesaurus, Application Domain, Technology Topic, Popular Technical Reports.
© 2024 PatSnap. All rights reserved.Legal|Privacy policy|Modern Slavery Act Transparency Statement|Sitemap|About US| Contact US: help@patsnap.com