User recommendation method
A recommendation method and user technology, applied in neural learning methods, data processing applications, special data processing applications, etc., can solve problems such as data sparseness, achieve the effect of improving robustness, solving data sparse problems, and enriching information
- Summary
- Abstract
- Description
- Claims
- Application Information
AI Technical Summary
Problems solved by technology
Method used
Image
Examples
Embodiment 1
[0029] The TDAE model is a model that integrates the user's rating information and the user's explicit trust relationship in the social network to predict and score the user's preference. figure 1 The flow chart of the user recommendation method provided in this application. Such as figure 1 shown, including the following steps:
[0030] Step S11: Determine the input data from the encoder.
[0031] Specifically, this application uses x∈R in the autoencoder N The vector of is used as input data. where x represents the input vector of the autoencoder, R N Indicates that the dimension of the vector x is N.
[0032] Step S12: Map the input data to the hidden layer of the autoencoder through an activation function. Among them, the specific representation of the hidden layer is as follows:
[0033] y=ρ(W T x+b)
[0034] Among them, y represents the result of the hidden layer, ρ represents the activation function, W represents the weight matrix of N×H dimensions, and W T Re...
Embodiment 2
[0065] The TDAE++ model is based on the TDAE model, which extracts the implicit trust relationship between users through the similarity measurement method, and then combines the explicit trust information and implicit trust information with scoring data to improve the quality of predictive scoring . Based on the first embodiment above, training model parameters on the data set further includes step S143: extracting implicit information from the data set through a similarity measurement method. On this basis, it is necessary to combine scoring data, explicit trust information and implicit information when predicting ratings.
[0066] Such as image 3 As shown, it specifically includes the following sub-steps:
[0067] Step S311: Measure the implicit trust relationship between users.
[0068] As an embodiment, this application uses similarity to measure the implicit trust relationship between users, which is specifically expressed as follows:
[0069]
[0070] Among them,...
Embodiment 3
[0083] This application selects PMF and DAE models as comparison models, and selects mean absolute error and root mean square error as evaluation criteria to measure the effect of the model.
PUM
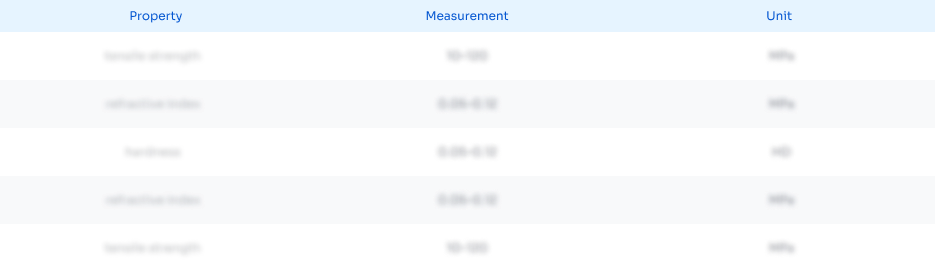
Abstract
Description
Claims
Application Information
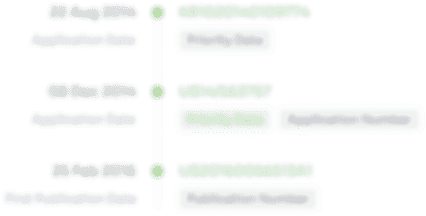
- R&D Engineer
- R&D Manager
- IP Professional
- Industry Leading Data Capabilities
- Powerful AI technology
- Patent DNA Extraction
Browse by: Latest US Patents, China's latest patents, Technical Efficacy Thesaurus, Application Domain, Technology Topic, Popular Technical Reports.
© 2024 PatSnap. All rights reserved.Legal|Privacy policy|Modern Slavery Act Transparency Statement|Sitemap|About US| Contact US: help@patsnap.com