Fast Hash Vehicle Retrieval Method Based on Multi-task Deep Learning
A deep learning, multi-task technology, applied in the field of intelligent transportation
- Summary
- Abstract
- Description
- Claims
- Application Information
AI Technical Summary
Problems solved by technology
Method used
Image
Examples
Embodiment Construction
[0097] The present invention will be further described below in conjunction with the accompanying drawings.
[0098] refer to Figure 1 to Figure 5 , a fast hash vehicle retrieval method based on multi-task deep learning, including:
[0099] The first step is to construct a multi-task deep convolutional neural network for deep learning and training recognition;
[0100] In the second step, the feature fusion method of piecewise compact hash code and instance features is adopted;
[0101] The third step is to use the local sensitive hash reordering algorithm;
[0102] The fourth step is to use the cross-modal retrieval method to realize vehicle retrieval.
[0103] In the first step described, a multi-task deep convolutional neural network for deep learning and training recognition, such as figure 1 As shown; Faster R-CNN is used as the basic network of the multi-task convolutional neural network; the front of the network is a 3×3 convolutional network, called conv1, followe...
PUM
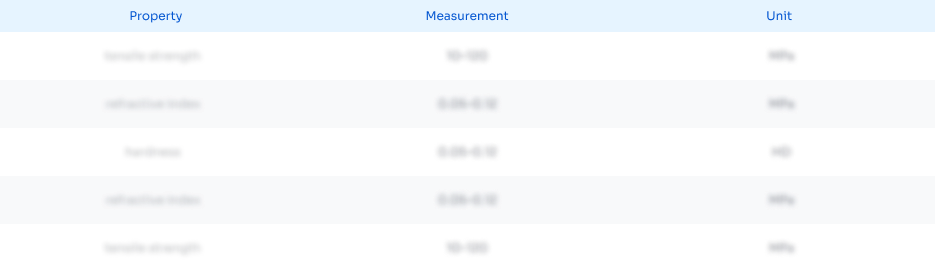
Abstract
Description
Claims
Application Information
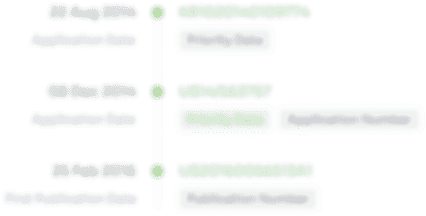
- Generate Ideas
- Intellectual Property
- Life Sciences
- Materials
- Tech Scout
- Unparalleled Data Quality
- Higher Quality Content
- 60% Fewer Hallucinations
Browse by: Latest US Patents, China's latest patents, Technical Efficacy Thesaurus, Application Domain, Technology Topic, Popular Technical Reports.
© 2025 PatSnap. All rights reserved.Legal|Privacy policy|Modern Slavery Act Transparency Statement|Sitemap|About US| Contact US: help@patsnap.com