Non-linear time-varying process fault monitoring method based on high efficiency recursion kernel principal component analysis
A nuclear principal component analysis and fault monitoring technology, applied in the direction of electrical testing/monitoring, can solve problems such as reducing the false alarm rate of faults, and achieve the effect of reducing the false alarm rate, low computing load, slow change, timely and accurate
- Summary
- Abstract
- Description
- Claims
- Application Information
AI Technical Summary
Problems solved by technology
Method used
Image
Examples
Embodiment Construction
[0050] The technical solutions of the present invention will be further described in detail below in conjunction with specific embodiments.
[0051] A kind of non-linear time-varying process fault monitoring method based on efficient recursive kernel principal component analysis proposed by the present invention comprises the following steps:
[0052] Step 1: Offline modeling. The normal operation data of the Tennessee Eastman chemical process was collected and standardized for the establishment of an offline nuclear principal component analysis model. The training data is mapped to the high-dimensional linear feature space through the radial basis kernel function, and then the eigenvalue and eigenvector of the sample covariance matrix are obtained by using the eigenvalue decomposition, and the offline model of the Tennessee Eastman chemical process is established; at the same time, with the help of the kernel Density estimation algorithm to determine the control limits of th...
PUM
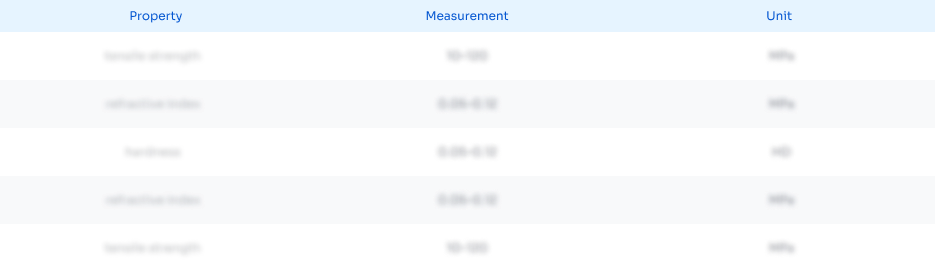
Abstract
Description
Claims
Application Information
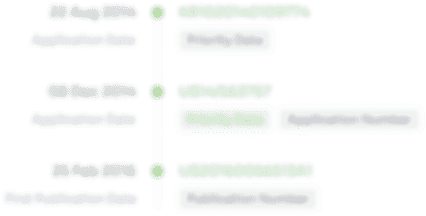
- R&D Engineer
- R&D Manager
- IP Professional
- Industry Leading Data Capabilities
- Powerful AI technology
- Patent DNA Extraction
Browse by: Latest US Patents, China's latest patents, Technical Efficacy Thesaurus, Application Domain, Technology Topic, Popular Technical Reports.
© 2024 PatSnap. All rights reserved.Legal|Privacy policy|Modern Slavery Act Transparency Statement|Sitemap|About US| Contact US: help@patsnap.com