Object detection method for remote sensing image based on convolutional neural network based on pruning strategy
A convolutional neural network and target detection technology, which is applied in the field of automatic construction of convolutional neural networks and remote sensing image target detection, can solve the problems of small target proportion, large remote sensing image images, and reduced network accuracy, and achieve redundant parameters. The effect of less, high accuracy and low missed detection rate
- Summary
- Abstract
- Description
- Claims
- Application Information
AI Technical Summary
Problems solved by technology
Method used
Image
Examples
Embodiment Construction
[0022] Step 1. Preprocess the target detection training data set so that the positive and negative ratios of the training set are balanced, and the output area extracts the training data set and the target classification training data set.
[0023] (1) Balanced samples: In each stochastic gradient descent iteration of training FRPN and AOCN, a small batch of samples with positive samples: negative samples = 1:3 (including all classes) is selected.
[0024] (2) Generate region extraction training data set
[0025] Using the category label and position label of the target detection training data set, the first part of the positive sample of the region extraction training data set is generated from the region marked by the position label in the target detection training data set; in order to make the region extraction network have the positioning ability, use the target detection The category label and position label of the training data set ensure that the IoU (Intersectionover ...
PUM
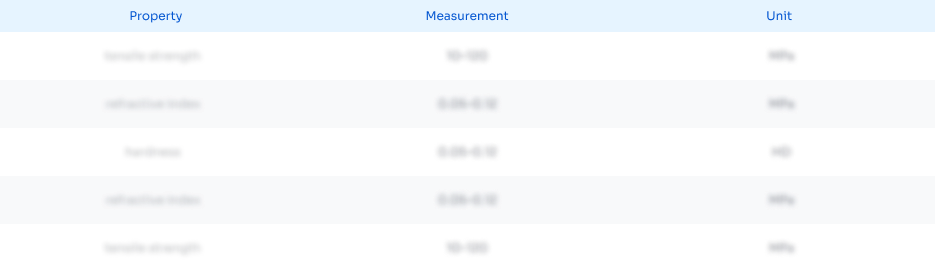
Abstract
Description
Claims
Application Information
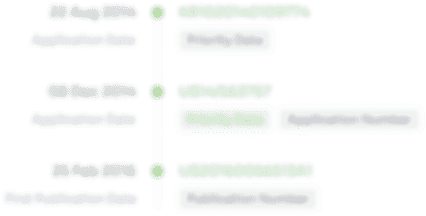
- R&D Engineer
- R&D Manager
- IP Professional
- Industry Leading Data Capabilities
- Powerful AI technology
- Patent DNA Extraction
Browse by: Latest US Patents, China's latest patents, Technical Efficacy Thesaurus, Application Domain, Technology Topic, Popular Technical Reports.
© 2024 PatSnap. All rights reserved.Legal|Privacy policy|Modern Slavery Act Transparency Statement|Sitemap|About US| Contact US: help@patsnap.com