EEMD-neural network based real-time data abnormal value detection method
A real-time data and neural network technology, applied in the field of data analysis, can solve problems such as unreliable real-time monitoring data
- Summary
- Abstract
- Description
- Claims
- Application Information
AI Technical Summary
Problems solved by technology
Method used
Image
Examples
Embodiment
[0102] In order to make the object, technical solution and advantages of the present invention clearer, the present invention will be further described in detail below in conjunction with the accompanying drawings and embodiments. It should be understood that the specific embodiments described here are only used to explain the present invention, not to limit the present invention.
[0103] Step 1: Identify research subjects and obtain data
[0104] Taking the daily water intake data of a waterworks B1 in North China as the research object. The daily water intake monitoring data comes from the national water resource management system database. The selected time range is two years from January 1, 2015 to December 31, 2016, a total of 731 days. The data of 365 days in 2015 is used as historical data, and the data of 366 days in 2016 is used as real-time data.
[0105] Step 2: Historical data outlier detection and correction
[0106] (2.1) Visualization of historical time ser...
PUM
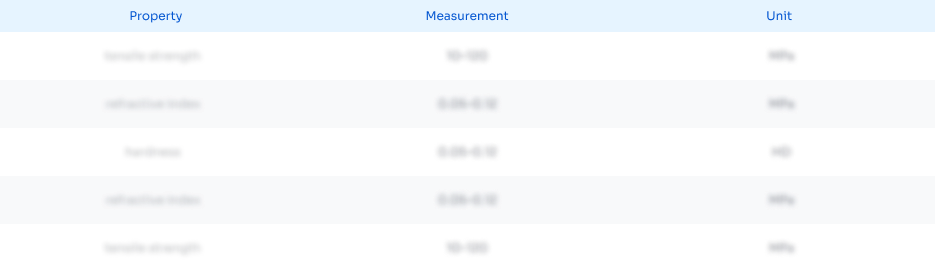
Abstract
Description
Claims
Application Information
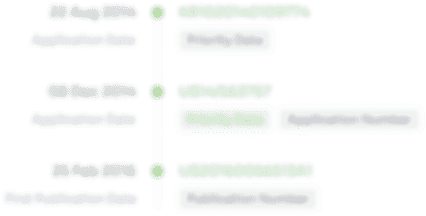
- R&D Engineer
- R&D Manager
- IP Professional
- Industry Leading Data Capabilities
- Powerful AI technology
- Patent DNA Extraction
Browse by: Latest US Patents, China's latest patents, Technical Efficacy Thesaurus, Application Domain, Technology Topic.
© 2024 PatSnap. All rights reserved.Legal|Privacy policy|Modern Slavery Act Transparency Statement|Sitemap