Human face age estimation method based on deep sparse representation
A technology of sparse representation and sparse representation coefficient, which is applied in the field of face age estimation based on deep sparse representation, which can solve the problem of lack of engineering application of massive age training samples, and achieve the effect of strengthening ability and reducing interference.
- Summary
- Abstract
- Description
- Claims
- Application Information
AI Technical Summary
Problems solved by technology
Method used
Image
Examples
Embodiment 1
[0070] A face age estimation method based on deep sparse representation proposed by the present invention mainly includes four steps: dictionary training, deep sparse representation model construction, feature extraction and age estimation.
[0071] Step 1: Dictionary Training
[0072] This step can be done offline in the background. First, collect face samples from 0-80 years old, each age as a class, each class contains 500 face images. Accordingly, the composition age face training set A=[A 1 , A 2 ,L,A 80 ],in is the jth sample face in the i-th category. Then, perform AAM, BIF and Gabor+LBP feature extraction on the face training set A. Finally, the discriminative dictionary learning method is used to train a complete dictionary D based on AAM, BIF and Gabor+LBP AAM ,D BIF ,D GL .
[0073] Step 2: Deep sparse representation model construction
[0074] Use the complete dictionary obtained in the previous step to build a deep sparse representation model, as shown...
Embodiment 2
[0086] A method for estimating the age of a human face based on depth sparse representation is the same as embodiment 1, wherein the method for discriminating dictionary learning in step 1 comprises the following steps:
[0087] (1a) Collect face samples from 0-80 years old, each age as a class, each class contains 500 face images. Accordingly, the composition age face training set A=[A 1 , A 2 ,L,A 80 ],in is the feature vector of the j-th sample in the i-th class. Assume that the training sample set A is represented by a linear combination of a complete dictionary D, and its sparse representation coefficient matrix is X. Then X can be rewritten as X=[X 1 , X 2 ,L,X 80 ], where X i for subset A i coefficient matrix.
[0088] (1b) In order to make the obtained complete dictionary D not only have good sparse reconstruction ability to sample set A, but also have strong discrimination and noise processing ability, the present invention constructs the following dictio...
Embodiment 3
[0109] A method for estimating the face age based on depth sparse representation is the same as embodiment 1-2, wherein the depth sparse representation model building method in step 2 includes the following steps:
[0110] (2a) The first layer design: Since the AAM feature integrates the face texture and shape information and is global, it is suitable for rough estimation of face age. Therefore, the first layer adopts AAM features. First, use the dictionary learning method introduced in step 1 to obtain the complete dictionary D AAM Perform sparse representation on the test face y to obtain the sparse representation coefficient x AAM :
[0111]
[0112] Among them, γ is a constant balance factor. Rewrite sparse representation coefficients Among them, the coefficient vector Corresponds to the sub-dictionary
[0113] Then, according to Define the residuals for each class:
[0114]
[0115] Among them, the first item is the reconstruction error item of the i-th...
PUM
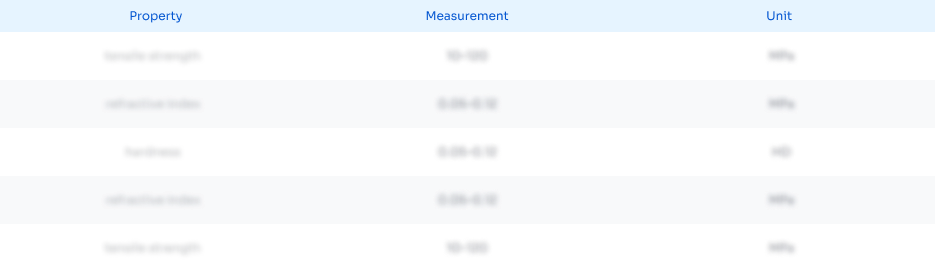
Abstract
Description
Claims
Application Information
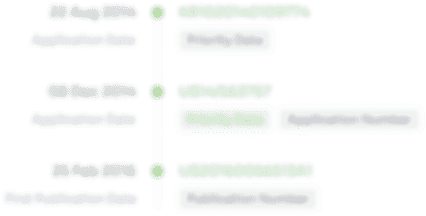
- Generate Ideas
- Intellectual Property
- Life Sciences
- Materials
- Tech Scout
- Unparalleled Data Quality
- Higher Quality Content
- 60% Fewer Hallucinations
Browse by: Latest US Patents, China's latest patents, Technical Efficacy Thesaurus, Application Domain, Technology Topic, Popular Technical Reports.
© 2025 PatSnap. All rights reserved.Legal|Privacy policy|Modern Slavery Act Transparency Statement|Sitemap|About US| Contact US: help@patsnap.com