Classifier chain local detection and mining algorithm
A mining algorithm and local detection technology, applied in computing, instruments, computing models, etc., can solve problems such as inability to perform distributed processing, load unloading methods that cannot obtain the best point-to-point effect, and cannot be realized
- Summary
- Abstract
- Description
- Claims
- Application Information
AI Technical Summary
Problems solved by technology
Method used
Image
Examples
Embodiment 1
[0048] Embodiment 1: as Figure 1 to Figure 5 As shown, a classifier chain local detection and mining algorithm, which includes: selecting a classifier a(n) within period n to maximize the expected reward E{r(n)}, the design idea is as follows:
[0049] S1: Each classifier m selects a classification function a in cycle n m (n)∈F m , a(n) represents the classifier chain in period n;
[0050] S2: After the data sample x(n) enters the system, the classification concept is generated through the classifier chain The final classification result can be expressed as
[0051] S3: At the end of the period, the total reward r(n) and total cost d(n) realized according to the true label z(n) will be displayed;
[0052] S4: If the accuracy and expected cost of each classification function for each classifier are known, the solution is:
[0053]
[0054] That is, the same classifier chain is selected in each time period to maximize the expected reward; where, a * Optimal classifi...
PUM
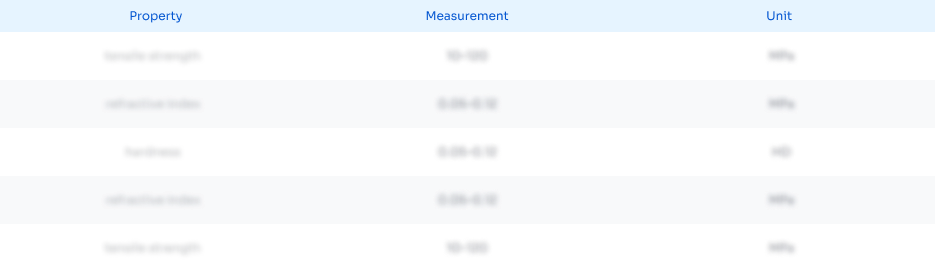
Abstract
Description
Claims
Application Information
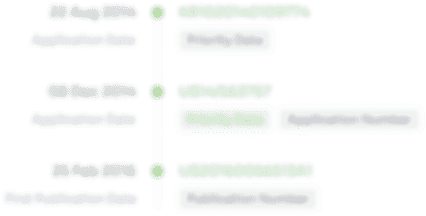
- R&D
- Intellectual Property
- Life Sciences
- Materials
- Tech Scout
- Unparalleled Data Quality
- Higher Quality Content
- 60% Fewer Hallucinations
Browse by: Latest US Patents, China's latest patents, Technical Efficacy Thesaurus, Application Domain, Technology Topic, Popular Technical Reports.
© 2025 PatSnap. All rights reserved.Legal|Privacy policy|Modern Slavery Act Transparency Statement|Sitemap|About US| Contact US: help@patsnap.com