Support vector machine wetland remote sensing classification method optimized by co-evolutionary algorithm
A support vector machine and co-evolution technology, applied in the field of support vector machine wetland remote sensing classification, can solve the problem that support vector machine and co-evolution algorithm have not yet appeared, achieve good execution efficiency and classification performance, improve evolution speed, low population diversity sexual effect
- Summary
- Abstract
- Description
- Claims
- Application Information
AI Technical Summary
Problems solved by technology
Method used
Image
Examples
specific Embodiment approach 1
[0042] 1. Basic principle and performance analysis
[0043] 1.1 Support Vector Machine Classification Principle
[0044] The mechanism of support vector machine (SVM) is to find an optimal classification hyperplane that meets the classification requirements, so that the hyperplane can maximize the blank area on both sides of the hyperplane while ensuring the classification accuracy; For example, given a training sample set (x i ,y i ), i=1,...,l; x∈R n , y∈{+1,-1}, the hyperplane is denoted as (w x)+b=0, in order to make the classification face all samples correctly classified and have a classification interval, it is required to meet the following constraints:
[0045] the y i [(w·x i )+b]≥1 i=1, 2, ..., l (1)
[0046] In the above formula (1), w represents the normal vector of the hyperplane, and b represents the bias;
[0047]It can be calculated that the classification interval is 2 / ||w||, so the problem of constructing the optimal hyperplane is transformed into fin...
PUM
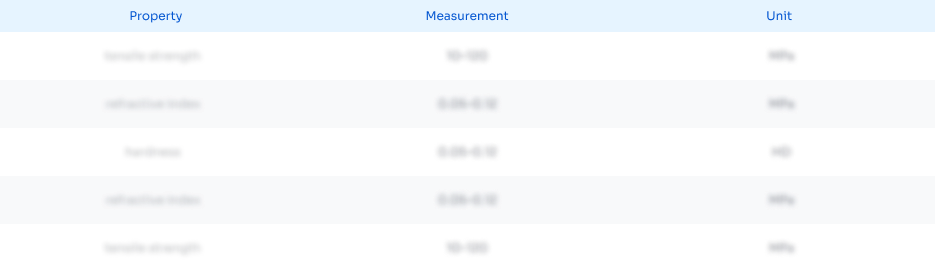
Abstract
Description
Claims
Application Information
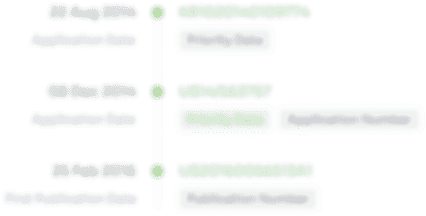
- R&D Engineer
- R&D Manager
- IP Professional
- Industry Leading Data Capabilities
- Powerful AI technology
- Patent DNA Extraction
Browse by: Latest US Patents, China's latest patents, Technical Efficacy Thesaurus, Application Domain, Technology Topic, Popular Technical Reports.
© 2024 PatSnap. All rights reserved.Legal|Privacy policy|Modern Slavery Act Transparency Statement|Sitemap|About US| Contact US: help@patsnap.com