Visual significance prediction method based on generative adversarial network
A prediction method, a remarkable technology, applied in the field of image analysis, can solve the problem of low stability
- Summary
- Abstract
- Description
- Claims
- Application Information
AI Technical Summary
Problems solved by technology
Method used
Image
Examples
Embodiment Construction
[0031] It should be noted that the embodiments in the application and the features in the embodiments can be combined with each other if there is no conflict. The present invention will be further described in detail below with reference to the drawings and specific embodiments.
[0032] figure 1 It is a system flow chart of the visual saliency prediction method based on generating confrontation network of the present invention. It mainly includes the architecture and training of the salient generation confrontation network (SalGAN) based on two deep convolutional neural network (DCNN) modules.
[0033] The filter weights in SalGAN have been trained by the perceptual loss caused by combining content and adversarial loss; the content loss follows the classic method, in which the predicted saliency map is compared with the corresponding saliency image from the calibrated real data in terms of pixels ; The adversarial loss depends on the discriminator, relative to the actual / synthetic...
PUM
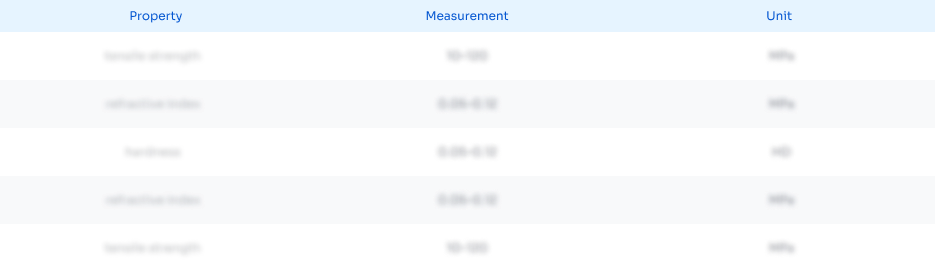
Abstract
Description
Claims
Application Information
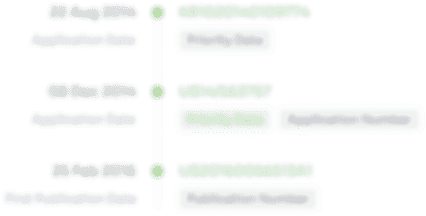
- R&D Engineer
- R&D Manager
- IP Professional
- Industry Leading Data Capabilities
- Powerful AI technology
- Patent DNA Extraction
Browse by: Latest US Patents, China's latest patents, Technical Efficacy Thesaurus, Application Domain, Technology Topic.
© 2024 PatSnap. All rights reserved.Legal|Privacy policy|Modern Slavery Act Transparency Statement|Sitemap