EEG (electroencephalogram) signal feature classification method based on ABC-SVM
A technology for classification of EEG signals and features, applied to instruments, character and pattern recognition, computer components, etc., can solve problems such as low stability, falling into local optimal values, low efficiency, etc., and achieve improved classification recognition rate, The effect of high accuracy
- Summary
- Abstract
- Description
- Claims
- Application Information
AI Technical Summary
Problems solved by technology
Method used
Image
Examples
Embodiment Construction
[0035] The present invention will be further described below in conjunction with accompanying drawing.
[0036] The present invention comprises the following steps:
[0037] Step 1. Use the CSP algorithm to extract the features of the EEG signal to obtain the sample feature vector f i ;
[0038] Step 2. utilize artificial bee colony algorithm to iteratively optimize the kernel function parameter and penalty factor of support vector machine;
[0039] Step 3. Use the optimal parameters optimized by the artificial bee colony algorithm to train the SVM classifier, and use the trained classifier to classify and predict the samples.
[0040] Wherein step 1, the EEG signal feature extraction obtains the feature vector and the specific steps are as follows:
[0041] The CSP algorithm is used to extract the features of the EEG signal, the number of channels of the experimental data is N, the number of sampling points of each channel is T, and the EEG data of one experiment is X n[N...
PUM
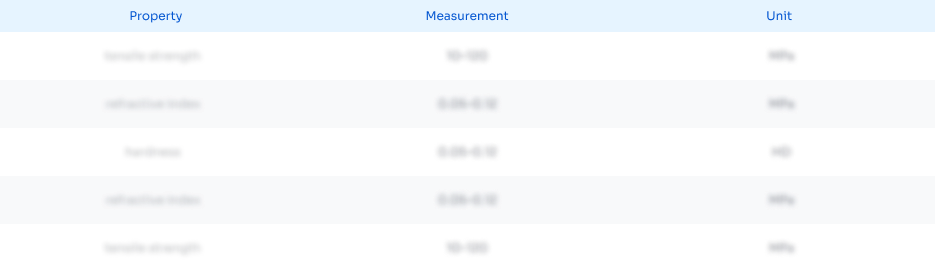
Abstract
Description
Claims
Application Information
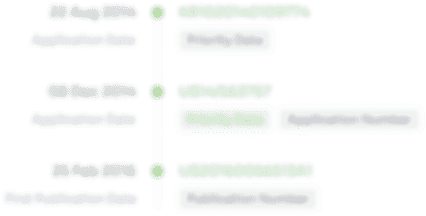
- R&D Engineer
- R&D Manager
- IP Professional
- Industry Leading Data Capabilities
- Powerful AI technology
- Patent DNA Extraction
Browse by: Latest US Patents, China's latest patents, Technical Efficacy Thesaurus, Application Domain, Technology Topic, Popular Technical Reports.
© 2024 PatSnap. All rights reserved.Legal|Privacy policy|Modern Slavery Act Transparency Statement|Sitemap|About US| Contact US: help@patsnap.com