Non-linear compression spectral imaging method based on hidden space worked example learning
A nonlinear compression and spectral imaging technology, applied in the field of image processing, can solve the problems of high time complexity and space complexity in dictionary learning, and achieve the effect of reducing time complexity and space complexity
- Summary
- Abstract
- Description
- Claims
- Application Information
AI Technical Summary
Problems solved by technology
Method used
Image
Examples
Embodiment Construction
[0029] refer to figure 1 , the specific implementation steps of the present invention are as follows:
[0030] Step 1. Build the training sample matrix.
[0031] Choose three groups n 1 ×n 2 ×n 3 Hyperspectral image of the hyperspectral image, randomly select n spectral segments except the 10th spectral segment to construct a size of w×n training sample matrix Y=[y 1 ,y 2 ,...,y j ,...,y n ], where w=n 1 ×n 2 , n 1 ×n 2 Indicates the size of the hyperspectral image, n 3 Indicates the number of total bands, y j Indicates the column vector drawn from the jth spectral segment, j=1,2,...,n, n is the number of training samples;
[0032] Step 2. Construct latent space training samples.
[0033] 2a) Randomly select t samples from n training samples to form a sub-sample matrix where t=20%n;
[0034] 2b) The selected kernel function is a polynomial kernel function k(x,y)=f k ()=(+s) d , find the simplified matrix of the kernel function: R=k(Y,Y R ),in s is the int...
PUM
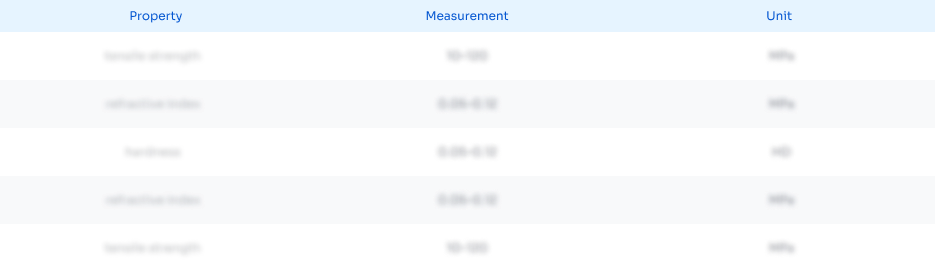
Abstract
Description
Claims
Application Information
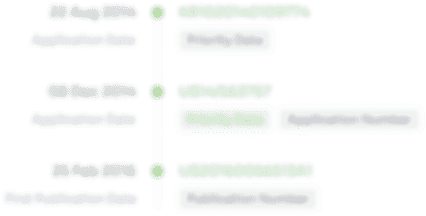
- R&D Engineer
- R&D Manager
- IP Professional
- Industry Leading Data Capabilities
- Powerful AI technology
- Patent DNA Extraction
Browse by: Latest US Patents, China's latest patents, Technical Efficacy Thesaurus, Application Domain, Technology Topic, Popular Technical Reports.
© 2024 PatSnap. All rights reserved.Legal|Privacy policy|Modern Slavery Act Transparency Statement|Sitemap|About US| Contact US: help@patsnap.com