Method for focus classification of sectional medical images by employing deep learning network
A deep learning network and medical imaging technology, applied in neural learning methods, biological neural network models, understanding of medical/anatomical patterns, etc., can solve the problem of inability to differentiate medical imaging lesions, and achieve the effect of improving work efficiency
- Summary
- Abstract
- Description
- Claims
- Application Information
AI Technical Summary
Problems solved by technology
Method used
Image
Examples
Embodiment Construction
[0037] In order to make the object, technical solution and advantages of the present invention clearer, the present invention will be further described in detail below in conjunction with the accompanying drawings and embodiments. It should be understood that the specific embodiments described here are only used to explain the present invention, not to limit the present invention.
[0038] In the embodiment of the present invention, the method for classifying lesions on tomographic medical images using a deep learning network, taking the tomographic medical images as MRI tomographic images as an example, mainly includes the following steps:
[0039] S1 case data labeling
[0040] Let case be X i , i∈[1,...,N], where N is the total number of cases, and each case has a label Y i , indicating whether it is PCA or BPH, say, if X i is PCA, then Y i = 1; if x i is BPH, then Y i = 2; in the case of MRI irradiation, each case has multi-layer image data, so the case can be repres...
PUM
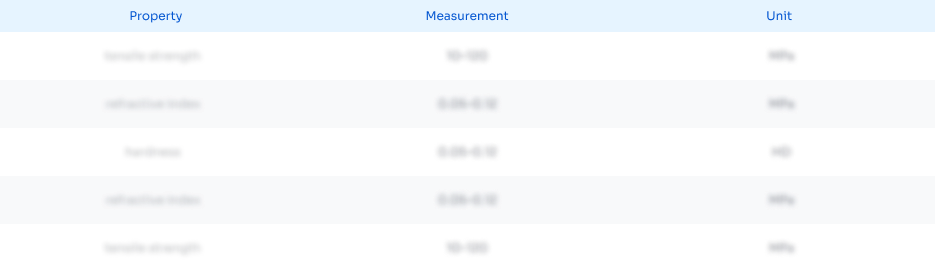
Abstract
Description
Claims
Application Information
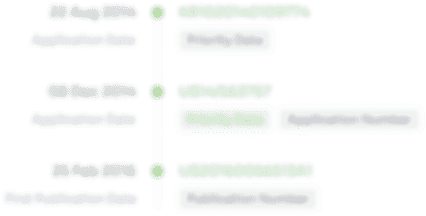
- Generate Ideas
- Intellectual Property
- Life Sciences
- Materials
- Tech Scout
- Unparalleled Data Quality
- Higher Quality Content
- 60% Fewer Hallucinations
Browse by: Latest US Patents, China's latest patents, Technical Efficacy Thesaurus, Application Domain, Technology Topic, Popular Technical Reports.
© 2025 PatSnap. All rights reserved.Legal|Privacy policy|Modern Slavery Act Transparency Statement|Sitemap|About US| Contact US: help@patsnap.com