Image object detection method
An object detection and image technology, applied in the fields of instruments, character and pattern recognition, computer components, etc., can solve the problems of increased ambiguity of data sets, unfavorable calculation speed, and poor detection performance.
- Summary
- Abstract
- Description
- Claims
- Application Information
AI Technical Summary
Problems solved by technology
Method used
Image
Examples
Embodiment Construction
[0022] figure 1 It is a flow chart of Embodiment 1 of the image object detection method of the present invention, figure 2 is a frame diagram of Embodiment 1 of the image object detection method of the present invention, as figure 1 As shown, the image object detection method of the present invention includes:
[0023] S101. Mark the multiple sample images according to the level of information, and obtain corresponding marked images;
[0024] Preferably, the information level includes strong labeling and weak labeling, and the multiple sample images are marked according to the information level, and obtaining the corresponding labeled images includes:
[0025] Add the category label and position label of the contained object to the sample image to obtain a strong label image; a strong label image refers to an image that knows the category and position of the object contained in the image;
[0026] or,
[0027] Add only the category annotations of the contained objects to ...
PUM
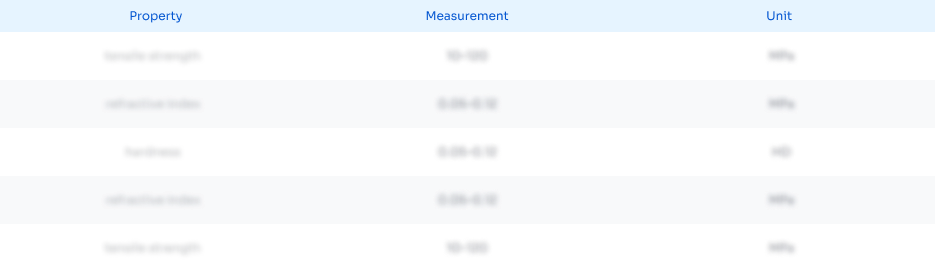
Abstract
Description
Claims
Application Information
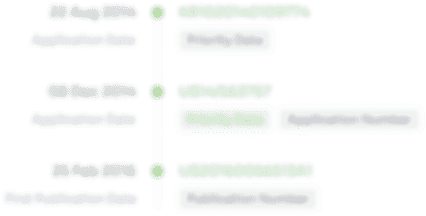
- R&D
- Intellectual Property
- Life Sciences
- Materials
- Tech Scout
- Unparalleled Data Quality
- Higher Quality Content
- 60% Fewer Hallucinations
Browse by: Latest US Patents, China's latest patents, Technical Efficacy Thesaurus, Application Domain, Technology Topic, Popular Technical Reports.
© 2025 PatSnap. All rights reserved.Legal|Privacy policy|Modern Slavery Act Transparency Statement|Sitemap|About US| Contact US: help@patsnap.com