Image quality testing method based on deep convolutional neural network
A deep convolution and image quality technology, applied in image analysis, image data processing, instruments, etc., can solve problems such as difficulty in designing discrimination, and achieve the effect of reducing impact, strong generalization, and improving accuracy
- Summary
- Abstract
- Description
- Claims
- Application Information
AI Technical Summary
Problems solved by technology
Method used
Image
Examples
Embodiment
[0035] Such as figure 1 As shown, the image quality testing method based on deep convolutional neural network of the present embodiment comprises the following steps:
[0036] (1) Establish a training sample set, and preprocess the pictures in the training sample set; the pictures in the sample set are selected from the image quality evaluation database of the Chinese University of Hong Kong (link: http: / / mmlab.ie.cuhk.edu.hk / datasets.html ), including 10,000 good-quality and poor-quality training pictures, and normalize all pictures to the size of 128*128; in order to remove the RGB (Red, Green, Blue) three Correlation between color channels, the present invention converts the data of RGB color space into HSV (Hue, Saturation, Value) color space, because in HSV color space, the correlation between each channel is small.
[0037] (2) Construct a deep convolutional neural network model: such as figure 2 As shown, the deep convolutional neural network includes sequentially c...
PUM
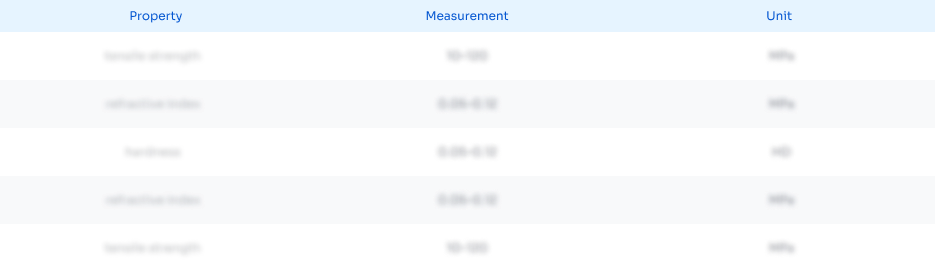
Abstract
Description
Claims
Application Information
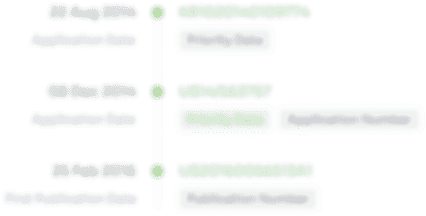
- R&D Engineer
- R&D Manager
- IP Professional
- Industry Leading Data Capabilities
- Powerful AI technology
- Patent DNA Extraction
Browse by: Latest US Patents, China's latest patents, Technical Efficacy Thesaurus, Application Domain, Technology Topic, Popular Technical Reports.
© 2024 PatSnap. All rights reserved.Legal|Privacy policy|Modern Slavery Act Transparency Statement|Sitemap|About US| Contact US: help@patsnap.com