Improved extreme learning machine combining learning thought of least square vector machine
An extreme learning machine and least squares technology, applied in the field of artificial intelligence, which can solve problems such as overfitting
- Summary
- Abstract
- Description
- Claims
- Application Information
AI Technical Summary
Problems solved by technology
Method used
Image
Examples
Embodiment 1
[0054] Example 1. Simulation data: "SinC"
[0055] "SinC" function expression:
[0056] y ( x ) = sin x / x , x ≠ 0 1 , x = 0
[0057] Data generation method: randomly generate 1000 training samples and 1000 test samples in the interval (-10, 10), and add random noise with a value range of [-0.2, 0.2] to all training samples, while the test data No noise; the experimental results of the three algorithms on the SinC dataset are shown in Table 1.
[0058] Table 1
[0059]
[0060] It can be obtained from Table 1 that because the ELM algorithm is b...
Embodiment 2
[0061] Example 2, Boston Housing data set
[0062] Boston Housing is a commonly used data set to measure the performance of regression algorithms, which can be obtained from the UCI database. It contains information about 506 commercial housing in the Boston Housing urban area, consisting of 12 continuous features, one discrete feature, and housing prices. The purpose of regression estimation is to predict the average price of houses through training on a part of the samples.
[0063] In the experiment, the sample set is randomly divided into two parts. The 256 sets of data in the training set are labeled samples, and the 250 sets of data in the test set are unlabeled samples. The experimental results of the three algorithms are shown in Table 2.
[0064] Table 2
[0065]
[0066] It can be seen from Table 2 that for the practical problem of multi-input and single-output in the Boston Housing dataset, the prediction errors of the ELM algorithm and the EOS-ELM algorithm are...
Embodiment 3
[0067] Example 3. Dissolved oxygen data set in actual fish farming
[0068] Dissolved oxygen is a very important water quality indicator in fish farming, and it plays an important role in the growth of fish. According to the actual situation, the experiment collected 360 sets of data from the Wuxi breeding base of the National Tilapia Industry Technology Research and Development Center as modeling data. The input data are pH value, temperature value, nitrate nitrogen value and ammonia nitrogen value, and the output data is dissolved oxygen value. The data is divided into 360 sets of 5-dimensional data through preprocessing, and the first 260 sets are selected as training data, and the last 100 sets are used as test data. The experimental results of the three algorithms are shown in Table 3.
[0069] table 3
[0070]
[0071] As can be seen from Table 3, the training errors of the three algorithms are very close, and the training errors of the first two algorithms are rel...
PUM
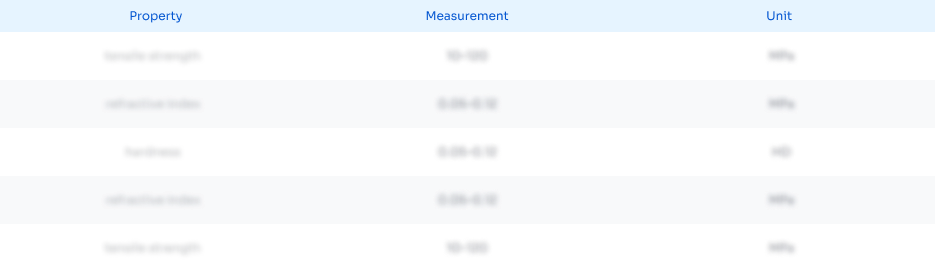
Abstract
Description
Claims
Application Information
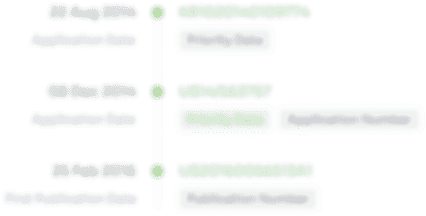
- Generate Ideas
- Intellectual Property
- Life Sciences
- Materials
- Tech Scout
- Unparalleled Data Quality
- Higher Quality Content
- 60% Fewer Hallucinations
Browse by: Latest US Patents, China's latest patents, Technical Efficacy Thesaurus, Application Domain, Technology Topic, Popular Technical Reports.
© 2025 PatSnap. All rights reserved.Legal|Privacy policy|Modern Slavery Act Transparency Statement|Sitemap|About US| Contact US: help@patsnap.com