Multi-modal joint representation learning method and system based on variational distillation
A learning method and multi-modal technology, applied in neural learning methods, character and pattern recognition, biological neural network models, etc., can solve the problem of lack of unified modal distillation method, and achieve the problem of forgetting, simple and effective, The effect of reducing information loss
- Summary
- Abstract
- Description
- Claims
- Application Information
AI Technical Summary
Problems solved by technology
Method used
Image
Examples
Embodiment 1
[0036] see figure 1 and 2 As shown, this embodiment provides a multimodal joint representation learning method based on variational distillation, including the following steps:
[0037]S1: Deploy a student model and a teacher model, the teacher model includes a text teacher model and an image teacher model, the student model includes a multimodal data unification module, and input raw multimodal data, wherein the original multimodal data includes Original text modal data and original image modal data, input the original text modal data and original image modal data into the multi-modal data unified module, obtain the text modal input and image modal input with the same input form, and compare the text modal data and image modal data. The modal input and the image modal input are normalized;
[0038] S2: the student model includes a modal joint representation module, and the normalized text modal input and image modal input are respectively input to the modal joint representa...
Embodiment 2
[0089] The following describes a multimodal joint representation learning system based on variational distillation disclosed in the second embodiment of the present invention. The multimodal joint representation learning system based on variational distillation described below is the same as the one based on The multimodal joint representation learning method of variational distillation can refer to each other correspondingly.
[0090] The second embodiment of the present invention discloses a multimodal joint representation learning system based on variational distillation, including:
[0091] A student model, the student model includes a multimodal data unified module and a modal joint representation module, and inputs original multimodal data, wherein the original multimodal data includes original text modal data and original image modal data, and the The original text modal data and the original image modal data are input to the multi-modal data unification module, the tex...
PUM
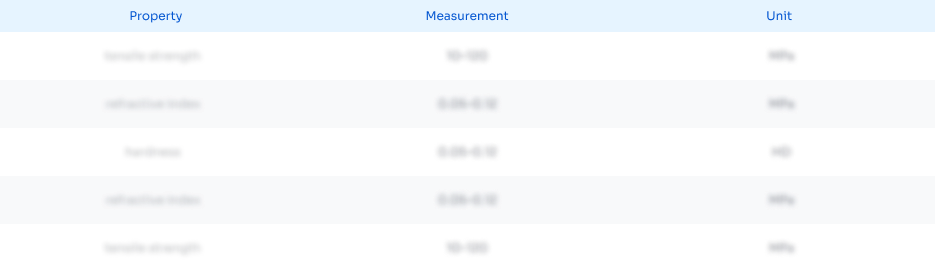
Abstract
Description
Claims
Application Information
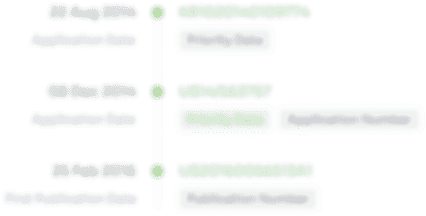
- R&D Engineer
- R&D Manager
- IP Professional
- Industry Leading Data Capabilities
- Powerful AI technology
- Patent DNA Extraction
Browse by: Latest US Patents, China's latest patents, Technical Efficacy Thesaurus, Application Domain, Technology Topic, Popular Technical Reports.
© 2024 PatSnap. All rights reserved.Legal|Privacy policy|Modern Slavery Act Transparency Statement|Sitemap|About US| Contact US: help@patsnap.com