Multi-task network model training method and system based on adaptive task weight
A network model and training method technology, applied in the field of computer vision, can solve problems such as inflexibility, disparity in learning effects, and difficulty in meeting actual needs, and achieve the effect of overcoming inflexibility and improving model performance.
- Summary
- Abstract
- Description
- Claims
- Application Information
AI Technical Summary
Problems solved by technology
Method used
Image
Examples
Embodiment Construction
[0061] The present invention will be further described below with reference to the accompanying drawings.
[0062] The method of the present invention is suitable for two task classification scenarios of image semantic segmentation and surface normal estimation, which are specifically:
[0063] 1. Select the dataset and perform preprocessing
[0064] NYU v2 is a dataset composed of RGB images of indoor scenes, in which there are 40 categories of semantic segmentation, such as beds, cabinets, clothes, books, etc. After using the standard training / validation segmentation, the training set has 795 images, and the validation set has 654 images. In addition, this dataset has pixel-level surface normal ground truth precomputed by depth markers.
[0065] 2. Build a multi-task model
[0066] The multi-task network model includes network models of multiple tasks; the network model of each task includes the ResNet backbone network and a specific task layer;
[0067] This model selec...
PUM
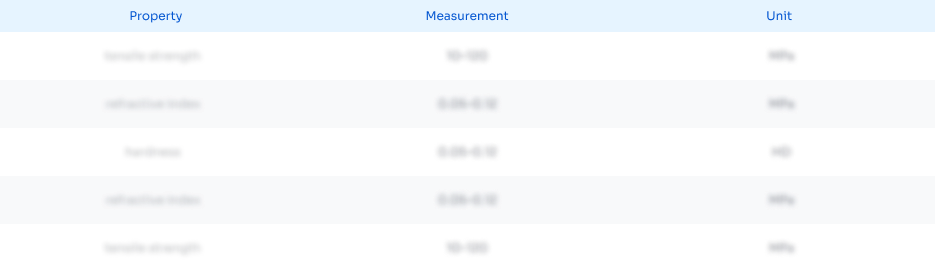
Abstract
Description
Claims
Application Information
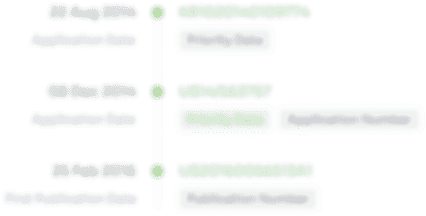
- R&D Engineer
- R&D Manager
- IP Professional
- Industry Leading Data Capabilities
- Powerful AI technology
- Patent DNA Extraction
Browse by: Latest US Patents, China's latest patents, Technical Efficacy Thesaurus, Application Domain, Technology Topic, Popular Technical Reports.
© 2024 PatSnap. All rights reserved.Legal|Privacy policy|Modern Slavery Act Transparency Statement|Sitemap|About US| Contact US: help@patsnap.com