Residual network model based on cross-stage local feature fusion strategy and training method of model
A technology of local features and network models, applied in biological neural network models, neural learning methods, computer components, etc., can solve problems such as network degradation, achieve the effect of improving accuracy, solving network degradation problems, and enhancing learning ability
- Summary
- Abstract
- Description
- Claims
- Application Information
AI Technical Summary
Problems solved by technology
Method used
Image
Examples
Embodiment 1
[0055] Assuming that there is already an optimal model for a research task that can just make the classification task 100% accurate, its network layer number is 10 layers. When we build the network model, this optimal model exists but is unknown, so we may set the number of network layers of 22 layers, then in fact, 12 layers of the designed network structure are redundant and useless. Unless the 12 layers become an identity map during model training, that is, the output through these 12 layers is exactly the same as the input, it is difficult for us to guarantee that the performance of the 22-layer network model is as good as the optimal 10-layer network model. Therefore, redundant network layer learning causes network degradation. In practical research, on the one hand, it is necessary to avoid network degradation caused by too many network layers, and on the other hand, it is necessary to deepen the network, make full use of processor computing power, and improve network pe...
Embodiment 2
[0093] The present invention also proposes a training method for a residual network model, which is used to train the residual network model based on the cross-stage local feature fusion strategy according to any one of claims 1 to 5. Before predicting the tool wear state, the residual network model of the local feature fusion strategy also includes training the model, and the training method includes:
[0094] acquiring a training data set, the training data set includes a tool monitoring signal with a training label, and the training label is the tool wear amount at the monitoring moment corresponding to the tool monitoring signal;
[0095] A residual network model based on a cross-stage local feature fusion strategy is trained using the training dataset.
[0096] The final output of the model is the same as the training label of the sample point (tool monitoring signal). At present, the training label commonly used in tool wear monitoring research is to encode the tool wea...
PUM
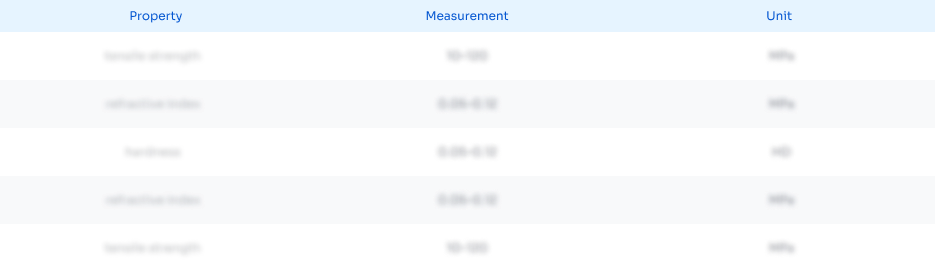
Abstract
Description
Claims
Application Information
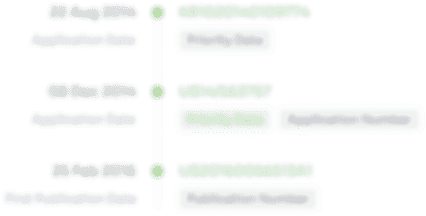
- R&D Engineer
- R&D Manager
- IP Professional
- Industry Leading Data Capabilities
- Powerful AI technology
- Patent DNA Extraction
Browse by: Latest US Patents, China's latest patents, Technical Efficacy Thesaurus, Application Domain, Technology Topic, Popular Technical Reports.
© 2024 PatSnap. All rights reserved.Legal|Privacy policy|Modern Slavery Act Transparency Statement|Sitemap|About US| Contact US: help@patsnap.com