Joint recommendation system based on joint learning framework
A recommendation system and framework technology, applied in the field of joint recommendation system based on the joint learning framework, can solve the problems of manpower and material resources
- Summary
- Abstract
- Description
- Claims
- Application Information
AI Technical Summary
Problems solved by technology
Method used
Image
Examples
Embodiment 1
[0035] see Figure 1 to Figure 2, a joint recommendation system based on a joint learning framework, including target domain input 1, main LSTM layer 2, main hidden layer 3, merge layer 4, main activation function layer 5, source domain input 6, auxiliary LSTM layer 7, The auxiliary hidden layer 8, the joint hidden layer 9, the auxiliary activation function layer 10, the auxiliary task output terminal 11 and the main task output terminal 12, the target field input terminal 1 receives the relevant data predicted by the target field, and generates the corresponding main task according to the relevant data. task, and send the main task to the main LSTM layer 2, the main LSTM layer 2 receives the main task, and makes the main task enter the LSTM network to participate in the judgment of the word segmentation NER model. At the same time, the main LSTM layer 2 is based on the target domain. Generate the main hidden layer 3, the target domain input 1 is connected to the target domain...
Embodiment 2
[0043] see Figure 1 to Figure 2 , a joint recommendation system based on a joint learning framework, including target domain input 1, main LSTM layer 2, main hidden layer 3, merge layer 4, main activation function layer 5, source domain input 6, auxiliary LSTM layer 7, The auxiliary hidden layer 8, the joint hidden layer 9, the auxiliary activation function layer 10, the auxiliary task output terminal 11 and the main task output terminal 12, the target field input terminal 1 receives the relevant data predicted by the target field, and generates the corresponding main task according to the relevant data. task, and send the main task to the main LSTM layer 2, the main LSTM layer 2 receives the main task, and makes the main task enter the LSTM network to participate in the judgment of the word segmentation NER model. At the same time, the main LSTM layer 2 is based on the target domain. Generate the main hidden layer 3, the target domain input 1 is connected to the target domai...
Embodiment 3
[0051] see Figure 1 to Figure 2 , a joint recommendation system based on a joint learning framework, including target domain input 1, main LSTM layer 2, main hidden layer 3, merge layer 4, main activation function layer 5, source domain input 6, auxiliary LSTM layer 7, The auxiliary hidden layer 8, the joint hidden layer 9, the auxiliary activation function layer 10, the auxiliary task output terminal 11 and the main task output terminal 12, the target field input terminal 1 receives the relevant data predicted by the target field, and generates the corresponding main task according to the relevant data. task, and send the main task to the main LSTM layer 2, the main LSTM layer 2 receives the main task, and makes the main task enter the LSTM network to participate in the judgment of the word segmentation NER model. At the same time, the main LSTM layer 2 is based on the target domain. Generate the main hidden layer 3, the target domain input 1 is connected to the target domai...
PUM
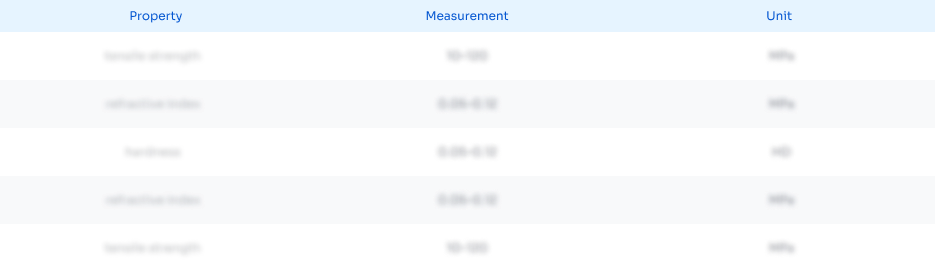
Abstract
Description
Claims
Application Information
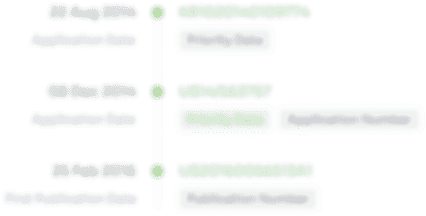
- R&D Engineer
- R&D Manager
- IP Professional
- Industry Leading Data Capabilities
- Powerful AI technology
- Patent DNA Extraction
Browse by: Latest US Patents, China's latest patents, Technical Efficacy Thesaurus, Application Domain, Technology Topic, Popular Technical Reports.
© 2024 PatSnap. All rights reserved.Legal|Privacy policy|Modern Slavery Act Transparency Statement|Sitemap|About US| Contact US: help@patsnap.com