Drug-target affinity prediction system based on graph convolutional neural network, computer equipment and storage medium
A convolutional neural network and neural network technology, applied in the field of drug repositioning prediction, to achieve the effect of reducing material cost, high cost and long development cycle
- Summary
- Abstract
- Description
- Claims
- Application Information
AI Technical Summary
Problems solved by technology
Method used
Image
Examples
Embodiment Construction
[0019] The technical solutions of the present invention are further described below in conjunction with specific embodiments.
[0020] like figure 1 As shown, the specific situation of the drug target affinity prediction system based on graph convolutional neural network described in this embodiment:
[0021] 1) Select two datasets, Davis and KIBA, and divide the datasets according to 80% as the training set and 20% as the test set.
[0022] 2) Using the RDKIT tool, convert SMILES into a two-dimensional matrix representation, convert SMILES into a vector form against the dictionary, encode the protein sequence, and save all data in a pt file.
[0023] 3) Call the data in the pt file, and input the two-dimensional matrix representation of SMILES into four kinds of graph convolutional neural networks to obtain 128-dimensional feature vectors. The graph convolutional neural network to which it belongs can be selected by the user, and the vector of SMILES can be selected by the u...
PUM
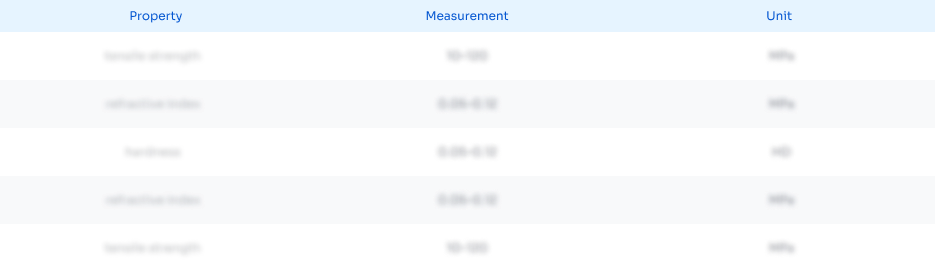
Abstract
Description
Claims
Application Information
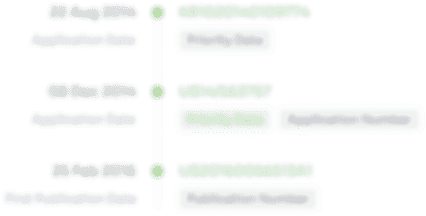
- R&D Engineer
- R&D Manager
- IP Professional
- Industry Leading Data Capabilities
- Powerful AI technology
- Patent DNA Extraction
Browse by: Latest US Patents, China's latest patents, Technical Efficacy Thesaurus, Application Domain, Technology Topic, Popular Technical Reports.
© 2024 PatSnap. All rights reserved.Legal|Privacy policy|Modern Slavery Act Transparency Statement|Sitemap|About US| Contact US: help@patsnap.com