Mechanical arm imitation learning method based on meta-action hierarchical generalization
A learning method and meta-action technology, applied in the field of imitation learning of robotic arms, can solve the problems of a large number of labor costs and high cost of expert teaching, and achieve the effects of improving accuracy, avoiding repeated training, and improving training efficiency
- Summary
- Abstract
- Description
- Claims
- Application Information
AI Technical Summary
Problems solved by technology
Method used
Image
Examples
Embodiment 1
[0038] Step S1: Move the manipulator by the hand of the expert, record the state of the manipulator joints with multiple heterogeneous sensors, and obtain the expert teaching data set, which is expressed as φ(s, a), where s represents the state data of each joint of the manipulator, including Spatial pose, moment, direction angle, etc.; a represents the robotic arm action mapped by the current state, recorded in a sparse matrix;
[0039] Step S2: Input the collected expert teaching data set φ(s, a) figure 1 In the generalization system in , the expert teaching dataset is decomposed by clustering, and the decomposed meta-action set is expressed as τ(A 1 , A 2 , …, A k ), where A 1 represents the first meta-action, and the subscript k represents the number of meta-actions included in the expert teaching;
[0040] Step S3: Calculate the weight of each meta action in the whole teaching action {π 1 , π 2 , …, π k }, where the weight coefficient π k One-to-one correspondence...
PUM
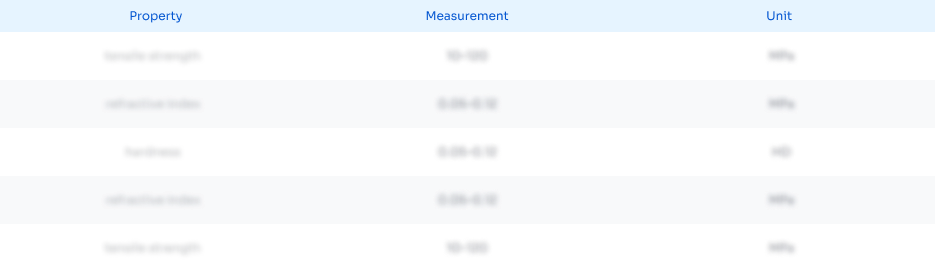
Abstract
Description
Claims
Application Information
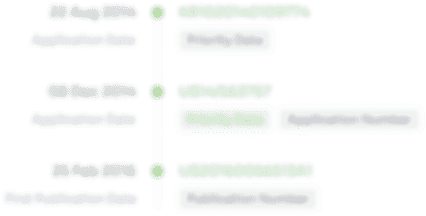
- R&D Engineer
- R&D Manager
- IP Professional
- Industry Leading Data Capabilities
- Powerful AI technology
- Patent DNA Extraction
Browse by: Latest US Patents, China's latest patents, Technical Efficacy Thesaurus, Application Domain, Technology Topic, Popular Technical Reports.
© 2024 PatSnap. All rights reserved.Legal|Privacy policy|Modern Slavery Act Transparency Statement|Sitemap|About US| Contact US: help@patsnap.com