Emotion classification continuous learning method based on iterative network combination and storage medium
A technology of emotion classification and learning method, which is applied in the field of text-based emotion classification, can solve problems such as task catastrophic forgetting and occupying large storage resources, so as to ensure continuous learning performance and resource cost advantages, save network resources, and improve network training efficiency Effect
- Summary
- Abstract
- Description
- Claims
- Application Information
AI Technical Summary
Problems solved by technology
Method used
Image
Examples
Embodiment Construction
[0063] The continuous learning method for emotion classification based on iterative network combination proposed by the present application includes the following steps: using BERT as the network model for the training data from multiple data sources to establish the original network 2; For new tasks; use BERT as the network model, adapt the original network 2 to the new task, and obtain the fine-tuning network 4; obtain the original combination parameters in the original network 2, and train the fine-tuning network 4 to obtain the fine-tuning combination parameters; freeze the original combination parameters and the fine-tuning combination Parameters; linearly combine the original network 2 and the fine-tuning network 4 to obtain the intermediate network 3; initialize the weight combination parameters of the intermediate network 3 to obtain the combination initialization parameters; obtain the original combination parameters and fine-tuning combination parameters in the interme...
PUM
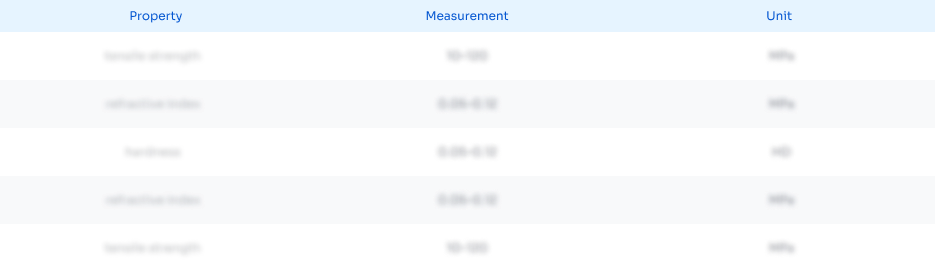
Abstract
Description
Claims
Application Information
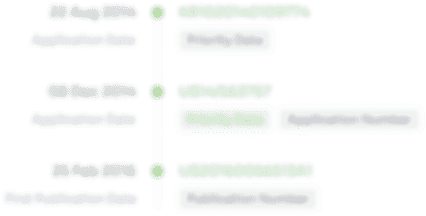
- R&D Engineer
- R&D Manager
- IP Professional
- Industry Leading Data Capabilities
- Powerful AI technology
- Patent DNA Extraction
Browse by: Latest US Patents, China's latest patents, Technical Efficacy Thesaurus, Application Domain, Technology Topic, Popular Technical Reports.
© 2024 PatSnap. All rights reserved.Legal|Privacy policy|Modern Slavery Act Transparency Statement|Sitemap|About US| Contact US: help@patsnap.com