Gating feature attention equivariant segmentation method based on weak supervised learning
A technology of attention and weak supervision, applied in the field of image processing, can solve the problems that the weak supervision segmentation network cannot be realized, and achieve the effect of improving classification accuracy and segmentation accuracy
- Summary
- Abstract
- Description
- Claims
- Application Information
AI Technical Summary
Problems solved by technology
Method used
Image
Examples
Embodiment Construction
[0043] The drawings constituting a part of the present invention are used to provide a further understanding of the present invention, and the schematic embodiments and descriptions of the present invention are used to explain the present invention, and do not constitute an improper limitation of the present invention.
[0044] figure 1 It is a schematic flow chart of the gated feature attention segmentation method based on weakly supervised learning proposed by the present invention, which can be specifically divided into three types: a twin classification network with equivariant constraints, a gated partial fusion module, and a cross feature attention module. module. The specific process steps are as follows:
[0045] First, build a ResNet38 classification network, send the image and its corresponding classification label into the network, and perform classification training through the classification loss function to obtain the category information of the image. In class...
PUM
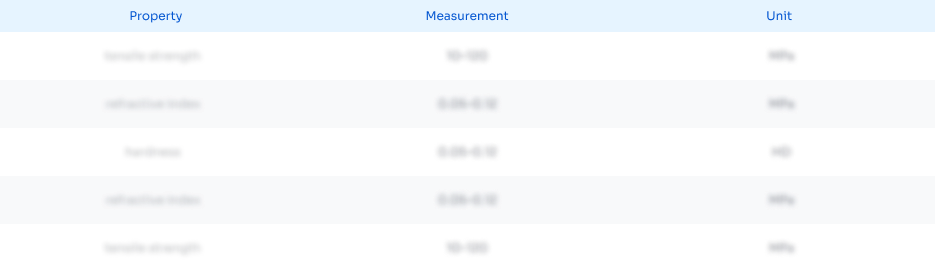
Abstract
Description
Claims
Application Information
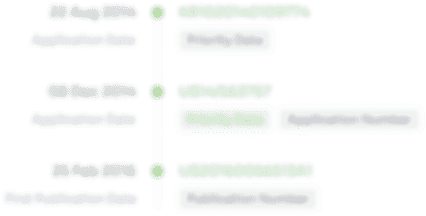
- R&D Engineer
- R&D Manager
- IP Professional
- Industry Leading Data Capabilities
- Powerful AI technology
- Patent DNA Extraction
Browse by: Latest US Patents, China's latest patents, Technical Efficacy Thesaurus, Application Domain, Technology Topic, Popular Technical Reports.
© 2024 PatSnap. All rights reserved.Legal|Privacy policy|Modern Slavery Act Transparency Statement|Sitemap|About US| Contact US: help@patsnap.com