New fault diagnosis method for rotating machinery based on deep confrontation convolutional neural network
A convolutional neural network and rotating machinery technology, applied in neural learning methods, biological neural network models, neural architectures, etc., can solve problems such as lack of new fault identification capabilities, inability to meet diagnostic needs, etc., to improve diversity and avoid artificial Feature extraction, the effect of reducing dependencies
- Summary
- Abstract
- Description
- Claims
- Application Information
AI Technical Summary
Problems solved by technology
Method used
Image
Examples
Embodiment Construction
[0048] In order to make the technical scheme and purpose of the present invention clearer, the present invention will be described in detail below in conjunction with the accompanying drawings and specific embodiments. It should be understood that the specific implementation steps described here are only used to better illustrate the application of the present invention. However, the technical features involved in the embodiments of the present invention are not limited thereto.
[0049] see figure 1 , the new method for fault diagnosis of rotating machinery based on deep anti-convolutional neural network (Domain Adversarial Convolutional Neural Network, DACNN) provided by the present invention comprises the following steps:
[0050] Step 1: Perform data collection and obtain a large number of source domain sample datasets {x s ,y s} and target domain sample dataset {x t}, the source domain sample data set includes the source domain data x s and its corresponding label y ...
PUM
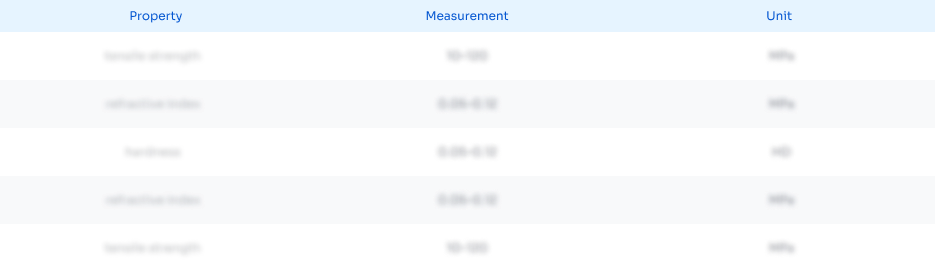
Abstract
Description
Claims
Application Information
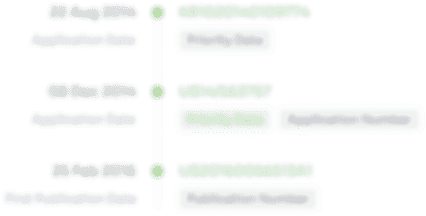
- R&D
- Intellectual Property
- Life Sciences
- Materials
- Tech Scout
- Unparalleled Data Quality
- Higher Quality Content
- 60% Fewer Hallucinations
Browse by: Latest US Patents, China's latest patents, Technical Efficacy Thesaurus, Application Domain, Technology Topic, Popular Technical Reports.
© 2025 PatSnap. All rights reserved.Legal|Privacy policy|Modern Slavery Act Transparency Statement|Sitemap|About US| Contact US: help@patsnap.com