Super-lens chromatic aberration recovery method based on deep learning
A technology of deep learning and recovery methods, applied in neural learning methods, image data processing, instruments, etc., can solve problems such as inability to solve chromatic aberration, poor image quality, reduced focus and imaging quality, etc., and achieve low-cost metal lens image chromatic aberration recovery , The effect of wide application and simple structure
- Summary
- Abstract
- Description
- Claims
- Application Information
AI Technical Summary
Problems solved by technology
Method used
Image
Examples
Embodiment Construction
[0032] The present invention will be described in detail below in conjunction with the accompanying drawings, but the present invention is not limited thereto.
[0033] Such as figure 1 As shown, first construct the superlens chromatic aberration recovery neural network: denote the hyperlens chromatic aberration image domain as X, and the standard image domain as Y, such as figure 2 As shown in the structural diagram of the superlens chromatic aberration restoration neural network, the superlens chromatic aberration restoration network consists of two X2Y generative adversarial neural networks and Y2X generative adversarial neural networks with the same structure, where the X2Y generative adversarial neural network is composed of the X2Y generator G Y and X2Y discriminator D Y Composition, Y2X Generative Adversarial Neural Network by Y2X Generator G X and Y2X discriminator D X constitute. G Y and G X Both consist of an encoder composed of three convolutional layers, a c...
PUM
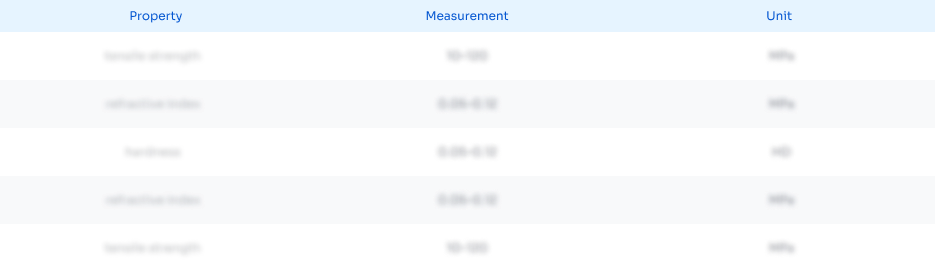
Abstract
Description
Claims
Application Information
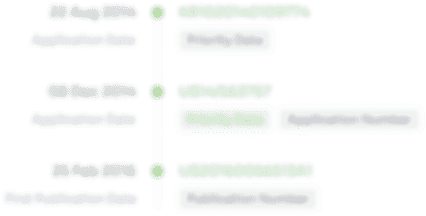
- R&D
- Intellectual Property
- Life Sciences
- Materials
- Tech Scout
- Unparalleled Data Quality
- Higher Quality Content
- 60% Fewer Hallucinations
Browse by: Latest US Patents, China's latest patents, Technical Efficacy Thesaurus, Application Domain, Technology Topic, Popular Technical Reports.
© 2025 PatSnap. All rights reserved.Legal|Privacy policy|Modern Slavery Act Transparency Statement|Sitemap|About US| Contact US: help@patsnap.com