Point cloud completion method based on local covariance optimization
A local covariance and point cloud technology, which is applied in the field of point cloud completion, can solve problems such as ignoring local structure and shape features, failing to restore local structure, and failing to complete the second task of point cloud. The effect of structural optimization
- Summary
- Abstract
- Description
- Claims
- Application Information
AI Technical Summary
Problems solved by technology
Method used
Image
Examples
Embodiment 1
[0043] The present invention provides a point cloud completion method based on local covariance optimization, such as figure 1 shown, including the following steps:
[0044] S1, data set acquisition, obtain the public data set ShapeNet, complete the construction of the point cloud data set described in the 3D model training, wherein the point cloud data set contains 55 different objects
[0045] S2, data preprocessing, perform surface sampling on each 3D model in the data set to obtain a complete point cloud, then simulate the process of shape loss to generate corresponding incomplete point clouds for each complete point cloud, and standardize all point cloud data, which is used for The preprocessed data set selects 16 common objects from 55 different objects in the data set, thus greatly reducing the amount of calculation;
[0046] Understandably, all point cloud data include complete point cloud and incomplete point cloud.
[0047] S3. The construction of neural network mo...
Embodiment 2
[0090] The difference between this embodiment and Embodiment 1 is that after the training of the neural network model CovNet is completed, the selected aircraft point cloud is selected to reduce the number of points of the incomplete point cloud from N=2048 to 35% when the missing ratio is 35%. 256. Such as Figure 5 As shown, the method proposed by the present invention still has excellent completion results.
Embodiment 3
[0092] The difference between this embodiment and Embodiment 1 is that after the training of the neural network model CovNet is completed, when the point quantity of the selected aircraft point cloud is fixed (N=2048) in the incomplete point cloud, the missing ratio is gradually increased from 0.25 to 0.75. Such as Figure 6 As shown, the method proposed by the present invention still has better completion results.
PUM
Property | Measurement | Unit |
---|---|---|
Learning rate | aaaaa | aaaaa |
Abstract
Description
Claims
Application Information
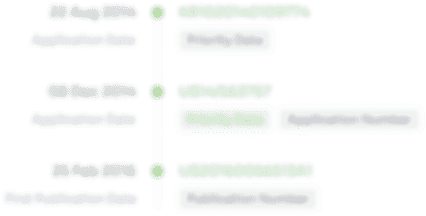
- R&D Engineer
- R&D Manager
- IP Professional
- Industry Leading Data Capabilities
- Powerful AI technology
- Patent DNA Extraction
Browse by: Latest US Patents, China's latest patents, Technical Efficacy Thesaurus, Application Domain, Technology Topic, Popular Technical Reports.
© 2024 PatSnap. All rights reserved.Legal|Privacy policy|Modern Slavery Act Transparency Statement|Sitemap|About US| Contact US: help@patsnap.com