Method for identifying impermeable surface of remote sensing shadow measurement repaired image
A recognition method and remote sensing image technology, applied in the field of remote sensing image non-permeable surface recognition, can solve problems such as parallel processing, difficulty in identifying urban non-permeable surfaces, and overestimation or underestimation of non-permeable surfaces.
- Summary
- Abstract
- Description
- Claims
- Application Information
AI Technical Summary
Problems solved by technology
Method used
Image
Examples
Embodiment Construction
[0084] The following describes the technical solution of the method for identifying non-permeable surfaces of remote sensing shading metering restoration images provided by this application in conjunction with the accompanying drawings, so that those skilled in the art can better understand this application and implement it.
[0085] Urban impermeable layer is one of the important indicators to measure the urban ecological environment and sustainable development. At present, with the acceleration of urbanization, the ratio of urban impermeable layer is increasing day by day, resulting in the reduction of urban green space and water body area, which has a negative impact on the urban ecological environment. It produces a series of negative impacts, such as urban heat island, waterlogging, non-point source pollution of water quality, etc., seriously affecting the lives of residents and the urban hydro-ecological structure. The acquisition of urban impervious surfaces by tradition...
PUM
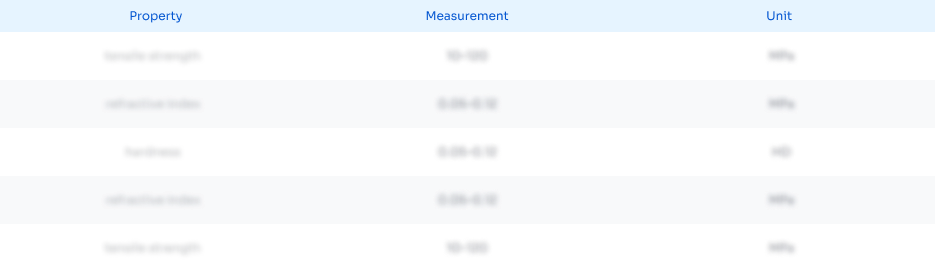
Abstract
Description
Claims
Application Information
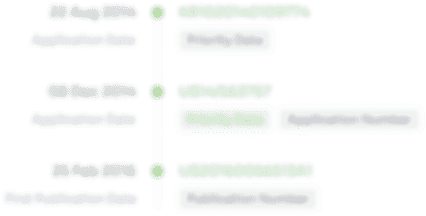
- Generate Ideas
- Intellectual Property
- Life Sciences
- Materials
- Tech Scout
- Unparalleled Data Quality
- Higher Quality Content
- 60% Fewer Hallucinations
Browse by: Latest US Patents, China's latest patents, Technical Efficacy Thesaurus, Application Domain, Technology Topic, Popular Technical Reports.
© 2025 PatSnap. All rights reserved.Legal|Privacy policy|Modern Slavery Act Transparency Statement|Sitemap|About US| Contact US: help@patsnap.com