Convolutional neural network architecture search method based on differentiable sampler and progressive learning
A technology of convolutional neural network and search method, which is applied in the field of convolutional neural network architecture search based on differentiable samplers and progressive learning, which can solve the problems of limited discretization error of supernetwork and loss of supernetwork, and achieve optimal probability distribution. function, the effect of reducing discretization error
- Summary
- Abstract
- Description
- Claims
- Application Information
AI Technical Summary
Problems solved by technology
Method used
Image
Examples
Embodiment 1
[0046] Please refer to figure 1 , a convolutional neural network architecture search method based on differentiable samplers and progressive learning, including the following steps:
[0047] S1, constructing a hypernetwork, architecture parameters of the hypernetwork, and a differentiable sampler;
[0048] S2, in a progressive learning manner, according to the architecture parameters, using the differentiable sampler to perform sampling optimization on the hypernetwork to obtain a desired network;
[0049] S3. Perform network retraining on the desired network until the desired network converges.
[0050] Compared with the prior art, the present invention directly samples and optimizes the constructed hypernetwork by using a differentiable sampler, which can change the optimization goal of the architecture search from optimizing the supernetwork to finding the optimal probability distribution function, minimizing the The expectation of the loss function of the network under t...
Embodiment 2
[0093] This embodiment is a further description made on the basis of Embodiment 1 in conjunction with specific parameter settings and with more specific examples, wherein:
[0094] In this embodiment, an architecture search is performed on the CIFAR-10 data set. Specifically, the CIFAR-10 dataset contains 60,000 pictures, of which 50,000 are training sets and 10,000 are verification sets. The picture resolution is 32×32 and the number of channels is 3.
[0095] For the CIFAR-10 data set: In this embodiment, the number of channels of the three-stage hypernetwork is predefined as [9,13,19]. ,5,3].
[0096] The forms of candidate neural operators include: 3×3 depthwise separable convolution, 5×5 depthwise separable convolution, 3×3 hole convolution, 5×5 hole convolution, 3×3 maximum pooling layer, 3× 3 average pooling layer, residual connection, zero operation.
[0097] The Dropouts of the residual connections in the three stages are [0, 0.4, 0.7] respectively.
[0098] The f...
Embodiment 3
[0107] A convolutional neural network architecture search system based on differentiable samplers and progressive learning, please refer to Figure 6, including a building block 1, a sampling optimization module 2 and a retraining module 3, the sampling optimization module 2 is respectively connected to the construction Module 1 and retraining module 3, in which:
[0108] The building block 1 is used to construct a hypernetwork, architectural parameters of the hypernetwork and a differentiable sampler;
[0109] The sampling optimization module 2 is used for progressive learning, according to the architecture parameters, using the differentiable sampler to perform sampling optimization on the hypernetwork to obtain a desired network;
[0110] The retraining module 3 is used to retrain the desired network until the desired network converges.
PUM
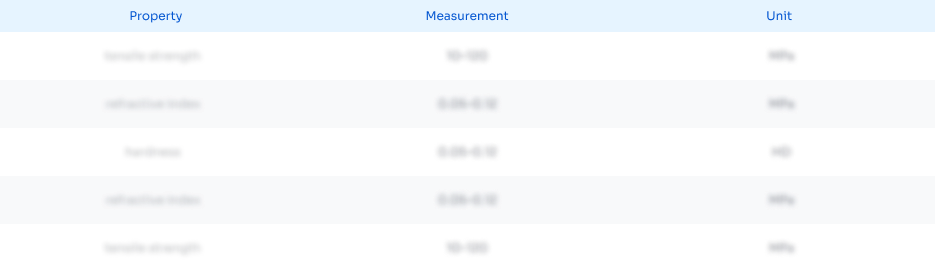
Abstract
Description
Claims
Application Information
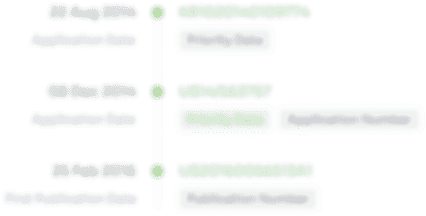
- R&D
- Intellectual Property
- Life Sciences
- Materials
- Tech Scout
- Unparalleled Data Quality
- Higher Quality Content
- 60% Fewer Hallucinations
Browse by: Latest US Patents, China's latest patents, Technical Efficacy Thesaurus, Application Domain, Technology Topic, Popular Technical Reports.
© 2025 PatSnap. All rights reserved.Legal|Privacy policy|Modern Slavery Act Transparency Statement|Sitemap|About US| Contact US: help@patsnap.com