Urban traffic flow prediction method based on Markov clustering graph attention network
A Markov clustering and urban transportation technology, applied in the field of transportation, can solve problems such as abandoning the overall structure and not being able to assign importance to adjacent nodes
- Summary
- Abstract
- Description
- Claims
- Application Information
AI Technical Summary
Problems solved by technology
Method used
Image
Examples
Embodiment Construction
[0055] Attached below figure 1 The present invention will be described in detail.
[0056] Such as figure 1 As shown, an urban traffic flow prediction method based on Markov cluster graph attention network includes the following steps:
[0057] Step 1: According to the historical traffic travel data, construct the structural information of the time series graph for the selected area, and obtain the corresponding flow matrix; the specific operation is divided into the following sub-steps:
[0058] 1.1) Structural map information: first divide the selected area into equidistant small-scale plots, use them as the nodes of the graph structure, set the number of nodes obtained as N, and mark them with 1-N serial numbers in turn; The selected area is divided into small-scale plots of 1km*1km, G={N}, where N={N 1 , N 2 ,...,N N ,} is a set of nodes; the calculation formula for dividing the selected area into equidistant small-scale plots is as follows:
[0059]
[0060]
...
PUM
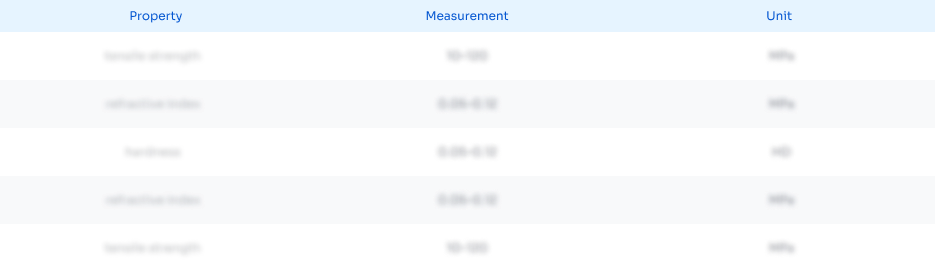
Abstract
Description
Claims
Application Information
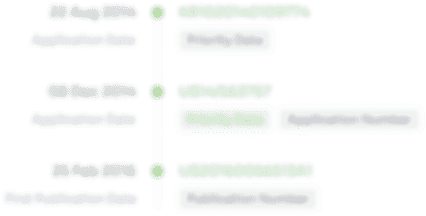
- R&D Engineer
- R&D Manager
- IP Professional
- Industry Leading Data Capabilities
- Powerful AI technology
- Patent DNA Extraction
Browse by: Latest US Patents, China's latest patents, Technical Efficacy Thesaurus, Application Domain, Technology Topic.
© 2024 PatSnap. All rights reserved.Legal|Privacy policy|Modern Slavery Act Transparency Statement|Sitemap