PU learning-based medical equipment performance index anomaly detection method and device
A technology for anomaly detection and medical equipment, applied in medical equipment, character and pattern recognition, instruments, etc., can solve problems such as low accuracy, inability to large-scale, diversified anomaly detection, and difficulty in implementation, to improve accuracy and reduce labeling The effect of workload
- Summary
- Abstract
- Description
- Claims
- Application Information
AI Technical Summary
Problems solved by technology
Method used
Image
Examples
Embodiment Construction
[0032] Embodiments of the present invention are described in detail below, examples of which are shown in the drawings, wherein the same or similar reference numerals designate the same or similar elements or elements having the same or similar functions throughout. The embodiments described below by referring to the figures are exemplary and are intended to explain the present invention and should not be construed as limiting the present invention.
[0033]It should be noted that, for a medical device, the KPI streams generated during the working process are numerous and have various modes. On the one hand, if a PU learning model is trained for each KPI stream, the workload of the overall labeling is very large; on the other hand, if a PU learning model is trained for all KPI streams, since different KPI streams have different patterns, different The most suitable anomaly detector and parameters for the KPI stream may be significantly different, so the model will suffer from ...
PUM
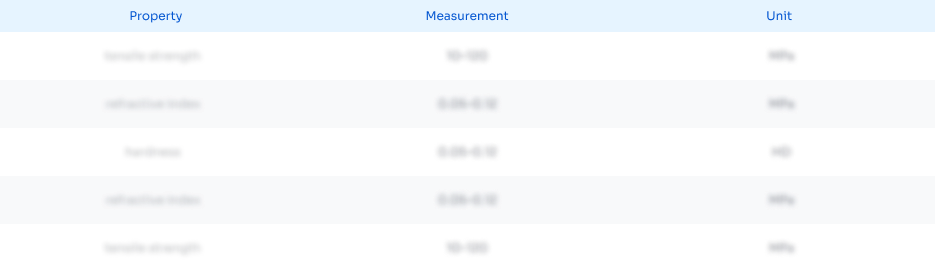
Abstract
Description
Claims
Application Information
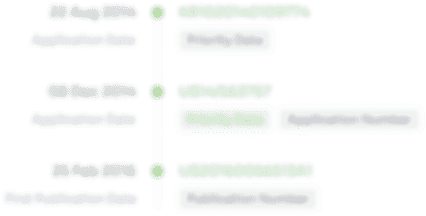
- R&D
- Intellectual Property
- Life Sciences
- Materials
- Tech Scout
- Unparalleled Data Quality
- Higher Quality Content
- 60% Fewer Hallucinations
Browse by: Latest US Patents, China's latest patents, Technical Efficacy Thesaurus, Application Domain, Technology Topic, Popular Technical Reports.
© 2025 PatSnap. All rights reserved.Legal|Privacy policy|Modern Slavery Act Transparency Statement|Sitemap|About US| Contact US: help@patsnap.com