Medical named entity recognition model training method, recognition method and federated learning system
A technology of named entity recognition and model training, which is applied in neural learning methods, character and pattern recognition, and biological neural network models. Risk of Data Leakage, Effects of Addressing Data Privacy
- Summary
- Abstract
- Description
- Claims
- Application Information
AI Technical Summary
Problems solved by technology
Method used
Image
Examples
Embodiment 1
[0058] like figure 1 As shown, the first aspect of the present invention provides a medical named entity recognition model training method. The method can be executed by a medical institution node, and the medical institution node can refer to an institution that performs model training based on a distributed server in the federated learning system, or can also refer to the distributed server itself in the federated learning system, including:
[0059] S101. Receive a global model for identifying a medical named entity sent by a central server.
[0060] An example of the overall federated learning framework of the present invention is figure 2 shown, including central server and distributed server. The distributed server corresponds to the medical named entity recognition system distributed in various medical institutions, and is used for model training and prediction locally, while the central server is responsible for initial model training, model issuance, gradient recov...
Embodiment 2
[0083] like Figure 4 As shown, the second aspect of the present invention provides a medical named entity recognition model training method. The method can be executed by the central server, and specifically includes:
[0084] S201. Distribute the global model for identifying medical named entities to each medical institution node in the federated learning system, so that each medical institution node trains the global model based on the respective local medical text annotation data, and calculates to obtain corresponding gradient data.
[0085] S202. Receive the gradient data respectively sent by each of the medical institution nodes, and train the global model based on each of the gradient data to obtain a new global model.
[0086] S203. If the new global model is currently converged, distribute the converged global model to each of the medical institution nodes in the federated learning system, so that each of the medical institution nodes is based on the corresponding ...
Embodiment 3
[0096] like Figure 5 As shown, the present invention provides, in a third aspect, a method for training a localized medical named entity recognition model. The method is executed by a medical institution node, and specifically includes:
[0097] S301. Obtain medical data.
[0098] In S301, the medical institution node can perform data cleaning, invalid data screening, and data format normalization processing on the medical data based on preset preprocessing rules, so as to further improve the execution efficiency and accuracy of S302.
[0099] S302. Input the medical data into a localized medical named entity recognition model, so that the localized medical named entity recognition model outputs a medical named entity recognition result corresponding to the medical data.
[0100] Wherein, the localized medical named entity recognition model is obtained in advance based on the medical named entity recognition model training method described in the first aspect.
[0101] Afte...
PUM
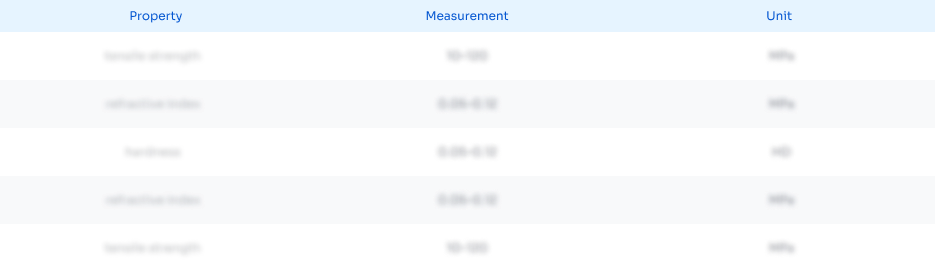
Abstract
Description
Claims
Application Information
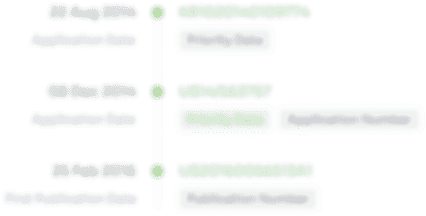
- Generate Ideas
- Intellectual Property
- Life Sciences
- Materials
- Tech Scout
- Unparalleled Data Quality
- Higher Quality Content
- 60% Fewer Hallucinations
Browse by: Latest US Patents, China's latest patents, Technical Efficacy Thesaurus, Application Domain, Technology Topic, Popular Technical Reports.
© 2025 PatSnap. All rights reserved.Legal|Privacy policy|Modern Slavery Act Transparency Statement|Sitemap|About US| Contact US: help@patsnap.com