Transformer winding deformation identification method based on LSTM neural network
A transformer winding and neural network technology, applied in the field of transformers, can solve problems such as lack of data, and achieve the effect of convenient construction of neural network and high fault identification efficiency
- Summary
- Abstract
- Description
- Claims
- Application Information
AI Technical Summary
Problems solved by technology
Method used
Image
Examples
Embodiment
[0155] The specific process is as figure 1As shown, it mainly includes the following steps:
[0156] (1) Carry out data cleaning and winding deformation fault calibration for transformer vibration data, such as figure 2 , whether this work is carried out smoothly will affect the convergence value and convergence speed of the loss value. The cleaning and calibration of samples account for 40% of the total workload, which determines whether the neural network results are convergent and overfitting, and finally it will be used as the LSTM neural network. Samples for model training.
[0157] (2) Carry out the design work of LSTM neural network, such as Figure 4 , Integrate the construction of LSTM neural network, including LSTM long-term short-term memory neural network for training vibration time-domain signals, and then conduct collective training with frequency-domain signals. The points of transformer vibration time-domain signals are 4096 points, and the number of LSTM ne...
PUM
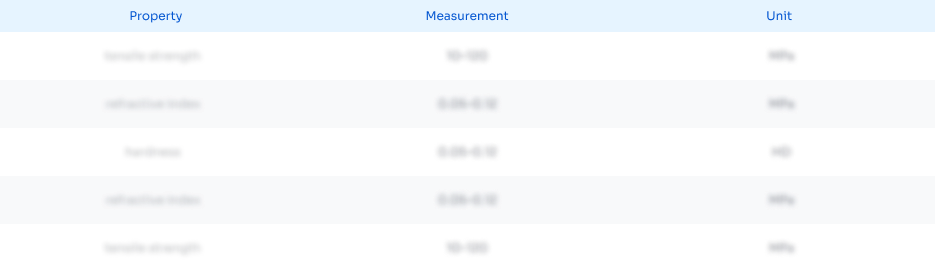
Abstract
Description
Claims
Application Information
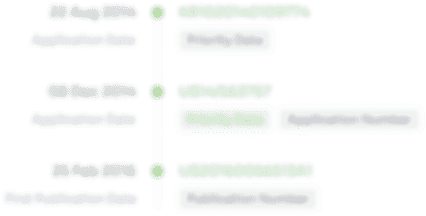
- R&D Engineer
- R&D Manager
- IP Professional
- Industry Leading Data Capabilities
- Powerful AI technology
- Patent DNA Extraction
Browse by: Latest US Patents, China's latest patents, Technical Efficacy Thesaurus, Application Domain, Technology Topic.
© 2024 PatSnap. All rights reserved.Legal|Privacy policy|Modern Slavery Act Transparency Statement|Sitemap