Model training and scene recognition method and device, equipment and medium
A scene recognition and model training technology, applied in the field of model training and scene recognition, can solve the problem of large impact on accuracy, and achieve the effect of improving accuracy and high extraction ability
- Summary
- Abstract
- Description
- Claims
- Application Information
AI Technical Summary
Problems solved by technology
Method used
Image
Examples
Embodiment 1
[0058] figure 1 A schematic diagram of the scene recognition model training process provided by the embodiment of the present invention, the process includes the following steps:
[0059] S101: Obtain parameters of the core feature extraction layer and the global information feature extraction layer through training using the first scene label of the sample image and the standard cross-entropy loss.
[0060] S102: Train the weight parameters of the LCS modules at each level according to the feature maps output by the LCS modules at each level and the loss values calculated pixel by pixel from the first scene label of the sample image.
[0061] S103: Using the first scene label of the sample image and the standard cross-entropy loss, train to obtain parameters of the fully-connected decision-making layer.
[0062] Wherein, the scene recognition model includes a core feature extraction layer, a global information feature extraction layer connected to the core feature extracti...
Embodiment 2
[0077] The core feature extraction layer includes a first-type grouping multi-receptive field residual convolution module and a second-type grouping multi-receptive field residual convolution module;
[0078] The multi-receptive field residual convolution module of the first type group includes a first group, a second group and a third group, the convolution sizes of the first group, the second group and the third group are different, and the first group The grouping, the second grouping and the third grouping include the residual calculation bypass structure; each grouping outputs feature maps through convolution operation and residual calculation, and the feature maps output by each grouping are concatenated in the channel dimension and channel shuffled, volume After the product is fused, it is output to the next module;
[0079] The multi-receptive field residual convolution module of the second grouping includes a fourth grouping, a fifth grouping and a sixth grouping, the...
Embodiment 3
[0082] The first scene label and the standard cross-entropy loss of the sample image are used to obtain the parameters of the core feature extraction layer and the global information feature extraction layer through training:
[0083] The feature maps of different levels in the core feature extraction layer are upsampled using deconvolution operations with different expansion factors, and the bilinear interpolation algorithm is used to align the number of channels in the channel dimension, and the feature maps of each level are added and merged channel by channel , the merged feature map group is convolutionally fused, and the global information feature vector is obtained by channel-by-channel global average pooling, the global information feature vector and the fully connected layer FC feature vector are spliced, and the standard cross-entropy loss is used to train The parameters of the core feature extraction layer and the global information feature extraction layer are obtai...
PUM
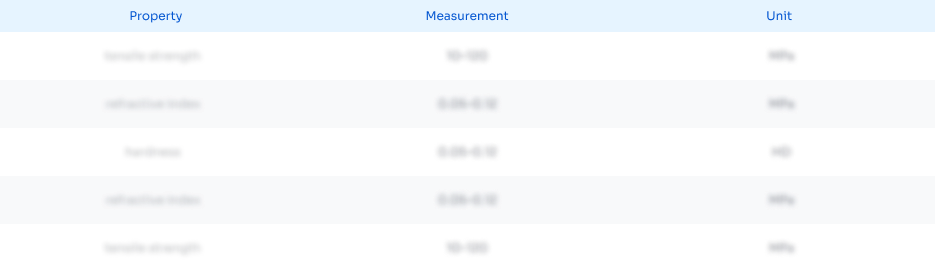
Abstract
Description
Claims
Application Information
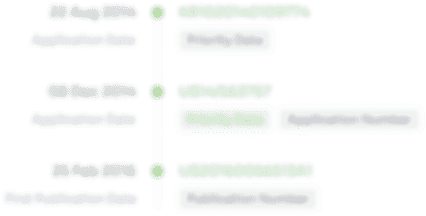
- Generate Ideas
- Intellectual Property
- Life Sciences
- Materials
- Tech Scout
- Unparalleled Data Quality
- Higher Quality Content
- 60% Fewer Hallucinations
Browse by: Latest US Patents, China's latest patents, Technical Efficacy Thesaurus, Application Domain, Technology Topic, Popular Technical Reports.
© 2025 PatSnap. All rights reserved.Legal|Privacy policy|Modern Slavery Act Transparency Statement|Sitemap|About US| Contact US: help@patsnap.com