Mechanical pump small sample fault diagnosis method based on WGAN-GP-C and metric learning
A technology of WGAN-GP-C and metric learning, which is applied to computer components, character and pattern recognition, instruments, etc., can solve problems such as difficulty in obtaining ideal results for algorithms, increasing difficulty of data analysis, and low accuracy of fault diagnosis , to achieve the effect of ensuring operation speed and classification performance, optimizing tree building time and search time, and high practical value
- Summary
- Abstract
- Description
- Claims
- Application Information
AI Technical Summary
Problems solved by technology
Method used
Image
Examples
Embodiment Construction
[0065] The technical solutions of the present invention will be further described below in conjunction with the accompanying drawings and embodiments. The following examples are only used to illustrate the technical solutions of the present invention more clearly, but not to limit the protection scope of the present application.
[0066] Such as figure 1 As shown, the mechanical pump small-sample fault diagnosis method based on WGAN-GP-C and metric learning of the present invention consists of data preprocessing, data enhancement based on WGAN-GP-C, classification algorithm based on metric learning and KNN, The model optimization consists of four parts to achieve accurate diagnosis of mechanical pump faults with a small sample background.
[0067] The mechanical pump small sample fault diagnosis method based on WGAN-GP-C and metric learning of the present invention, the specific steps are as follows:
[0068] (1) Data preprocessing design;
[0069] The original data was rea...
PUM
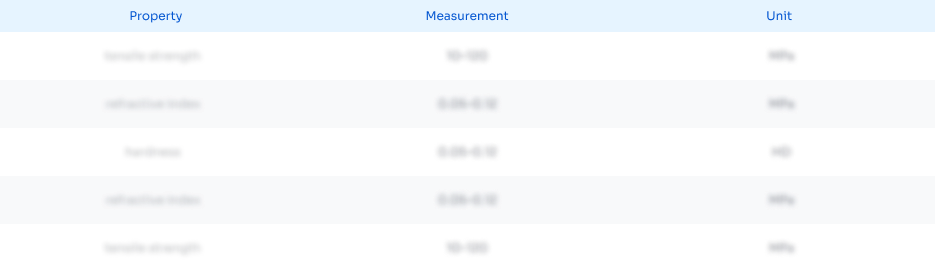
Abstract
Description
Claims
Application Information
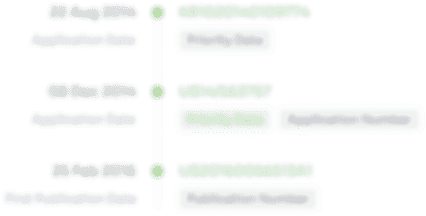
- R&D
- Intellectual Property
- Life Sciences
- Materials
- Tech Scout
- Unparalleled Data Quality
- Higher Quality Content
- 60% Fewer Hallucinations
Browse by: Latest US Patents, China's latest patents, Technical Efficacy Thesaurus, Application Domain, Technology Topic, Popular Technical Reports.
© 2025 PatSnap. All rights reserved.Legal|Privacy policy|Modern Slavery Act Transparency Statement|Sitemap|About US| Contact US: help@patsnap.com