Compressed sensing magnetic resonance reconstruction method of AttISTANet based on attention mechanism
A compressed sensing and attention technology, applied in neural learning methods, 2D image generation, image data processing, etc., can solve problems such as lack of attention, indistinguishable learning features, etc., and achieve good generalization ability, PSNR value and SSIM The effect of increasing the value
- Summary
- Abstract
- Description
- Claims
- Application Information
AI Technical Summary
Problems solved by technology
Method used
Image
Examples
specific Embodiment approach 1
[0052] A kind of AttISTANet compressed sensing magnetic resonance reconstruction method based on attention mechanism described in the present embodiment is characterized in that, the method comprises the steps:
[0053] Step 1: ISTANet + Construction of network structure;
[0054] Added H(·) and L(·) operators on the basis of ISTANet, where H k ( ) and L k ( ) is a 1×1 convolution kernel, which are linear operators for increasing the number of channels and reducing the number of channels, respectively, and are respectively linear operators for increasing the number of channels and reducing the number of channels, and use the residual structure to reduce the difficulty of network training ;
[0055] Step 2: the MRI reconstruction model construction based on the attention module;
[0056] on ISTANet + The attention module is introduced into the network, and in each reconstructed image x k The improved channel attention module and spatial attention module were added before,...
specific Embodiment approach 2
[0061] This embodiment is a further description of the AttISTANet compressed sensing magnetic resonance reconstruction method based on the attention mechanism described in the first embodiment. The specific process of the first step is: on the basis of ISTANet, H(·) and L(·) operator, where H k ( ) and L k ( ) is a 1×1 convolution kernel, which are linear operators for increasing the number of channels and reducing the number of channels, respectively, and are respectively linear operators for increasing the number of channels and reducing the number of channels, and use the residual structure to reduce the difficulty of network training , ISTANet + The iteration of the kth stage in the method is as follows:
[0062] r k =x k-1 -ρ k Φ T (Φx k-1 -y) (1)
[0063] x k = L k (F k ) T (soft(F k (H k (r k )), θ k ))+r k (2)
[0064] In reconstructing MRI images, the K-space data features used are scattered in different areas, ADMMNet, ISTANet + The equal processi...
specific Embodiment approach 3
[0065] This embodiment is a further description of the AttISTANet compressed sensing magnetic resonance reconstruction method based on the attention mechanism described in the first embodiment, and the specific process of the second step is: in the ISTANet + The network introduces different attention modules, and in each reconstructed image x k reconstruction and intermediate variable r k Added the improved channel attention module and spatial attention module before, and used the attention module to recalibrate the channel and spatial features of the original K-space data features. After passing the channel attention module and spatial attention module, the expression is:
[0066] C k = L k (F k ) T (soft(F k (H k (r k )), θ k )) (3)
[0067] a k =sigmoid(relu(w(C k ))) (4)
[0068] the s k =sigmoid(f(a k )) (5)
[0069] x k =C k ×s k + r k (6)
PUM
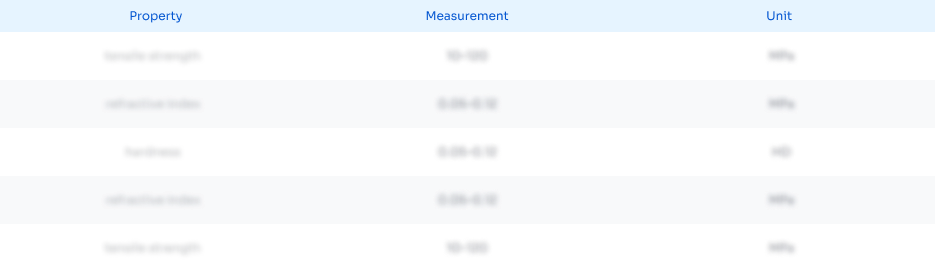
Abstract
Description
Claims
Application Information
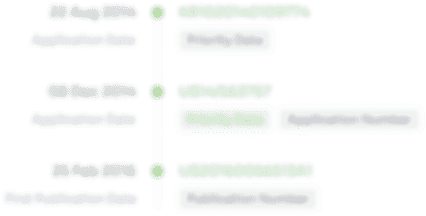
- R&D Engineer
- R&D Manager
- IP Professional
- Industry Leading Data Capabilities
- Powerful AI technology
- Patent DNA Extraction
Browse by: Latest US Patents, China's latest patents, Technical Efficacy Thesaurus, Application Domain, Technology Topic, Popular Technical Reports.
© 2024 PatSnap. All rights reserved.Legal|Privacy policy|Modern Slavery Act Transparency Statement|Sitemap|About US| Contact US: help@patsnap.com