Text mining method for heterogeneous graph conversion based on meta-structure learning
A text mining and heterogeneous graph technology, applied in neural learning methods, unstructured text data retrieval, data mining, etc., can solve problems such as mining of difficult semantic patterns, loss of semantic information, and complex real relationships
- Summary
- Abstract
- Description
- Claims
- Application Information
AI Technical Summary
Problems solved by technology
Method used
Image
Examples
Embodiment Construction
[0051] In order to make the purpose, technical solution and advantages of the present invention clearer, the present invention will be further elaborated below in conjunction with the accompanying drawings.
[0052] In this example, see figure 1 and 2 As shown, the present invention proposes a text mining method for heterogeneous graph conversion based on meta-structure learning, including steps:
[0053] S10, for the text data, extracting information in the text to construct a heterogeneous information network graph;
[0054] S20, using the graph conversion layer to obtain meta-paths to capture the relationship between nodes;
[0055] S30, extracting the metagraph structure by establishing a channel-type Hadamard product module, so as to capture multiple interactions between nodes;
[0056] S40, using a graph convolutional network for the extracted meta-structure including meta-paths and meta-graphs, to generate node embeddings;
[0057] S50, using the obtained node embed...
PUM
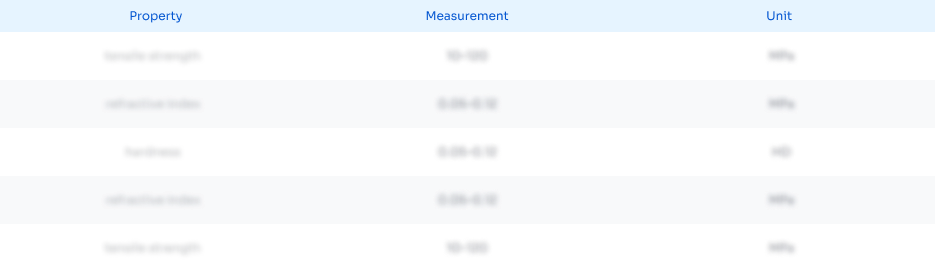
Abstract
Description
Claims
Application Information
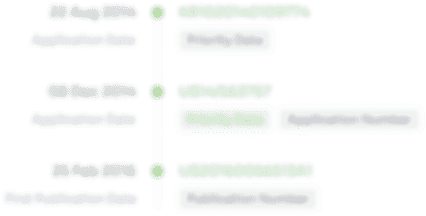
- R&D Engineer
- R&D Manager
- IP Professional
- Industry Leading Data Capabilities
- Powerful AI technology
- Patent DNA Extraction
Browse by: Latest US Patents, China's latest patents, Technical Efficacy Thesaurus, Application Domain, Technology Topic, Popular Technical Reports.
© 2024 PatSnap. All rights reserved.Legal|Privacy policy|Modern Slavery Act Transparency Statement|Sitemap|About US| Contact US: help@patsnap.com