Turbomachinery aerodynamic performance-blade load optimization method based on machine learning
A turbomachinery and machine learning technology, applied in the direction of neural learning methods, based on specific mathematical models, design optimization/simulation, etc., can solve the problems of difficult access to turbomachinery flow field information, increased calculation costs and time-consuming, and poor physical interpretation and other issues, to achieve the effect of no need for manual intervention, reduce cost and time-consuming, and reduce design cycle
- Summary
- Abstract
- Description
- Claims
- Application Information
AI Technical Summary
Problems solved by technology
Method used
Image
Examples
Embodiment Construction
[0061] The present invention will be described in further detail below in conjunction with the accompanying drawings and specific embodiments.
[0062] see figure 1 , the machine learning-based turbomachinery aerodynamic performance-blade load optimization method provided by the present invention comprises the following steps:
[0063]S1: Determine the working fluid of the turbomachinery, parameterize the turbomachinery to obtain the input variable x and the optimization target y=f(x) of the optimization process, and determine the empirical design space of the input variable x (that is, the value range and constraint relationship) . Among them, the input variable x includes the airflow angle α along the blade, the meridian surface shape z, and the blade thickness d along the distribution of turbomachinery geometric parameters; the optimization target y is efficiency, power, blade load or any aerodynamic parameter.
[0064] refer to image 3 , using the fourth-order Bezier c...
PUM
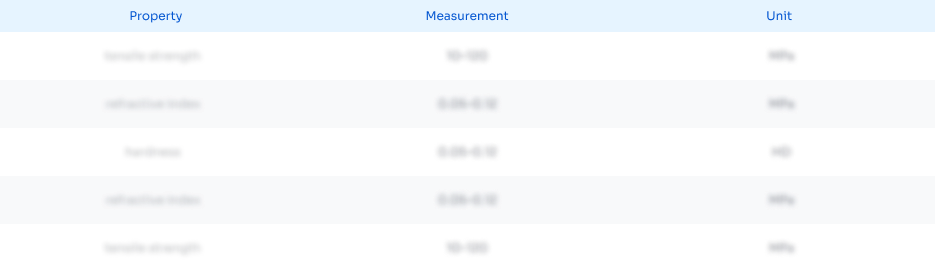
Abstract
Description
Claims
Application Information
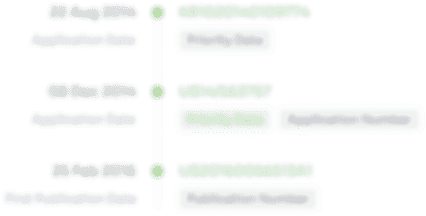
- R&D Engineer
- R&D Manager
- IP Professional
- Industry Leading Data Capabilities
- Powerful AI technology
- Patent DNA Extraction
Browse by: Latest US Patents, China's latest patents, Technical Efficacy Thesaurus, Application Domain, Technology Topic, Popular Technical Reports.
© 2024 PatSnap. All rights reserved.Legal|Privacy policy|Modern Slavery Act Transparency Statement|Sitemap|About US| Contact US: help@patsnap.com