Wind power prediction method and system and computer readable medium
A technology of wind power forecasting and forecasting methods, applied in the field of computer-readable media and wind power forecasting, can solve problems such as time-consuming, lack of theoretical guidance, and failure to consider original data information analysis, etc., to improve generalization ability and accuracy , Improve the effect of learning speed and generalization ability
- Summary
- Abstract
- Description
- Claims
- Application Information
AI Technical Summary
Problems solved by technology
Method used
Image
Examples
Embodiment
[0038] Such as figure 1 As shown, a wind power prediction method, the prediction method specifically includes the following steps:
[0039] S1. Collect wind power sample data, and divide the wind power sample data into a training set and a test set;
[0040] S2. Calculate the cluster center of the training set by fuzzy C-means clustering (FCM), calculate the Euclidean distance from the sample point in the test set to the cluster center and match the corresponding category;
[0041] S3. The clustered wind power sample data is decomposed and denoised by the variational mode decomposition (VMD) algorithm, and the reconstructed wind power sample data is input into multiple extreme learning machine prediction models for training;
[0042] S4. Select and optimize the kernel function in the extreme learning machine (SVM) prediction model through the particle swarm optimization (PSO) algorithm, obtain the optimized extreme learning machine prediction model, obtain wind power real-tim...
PUM
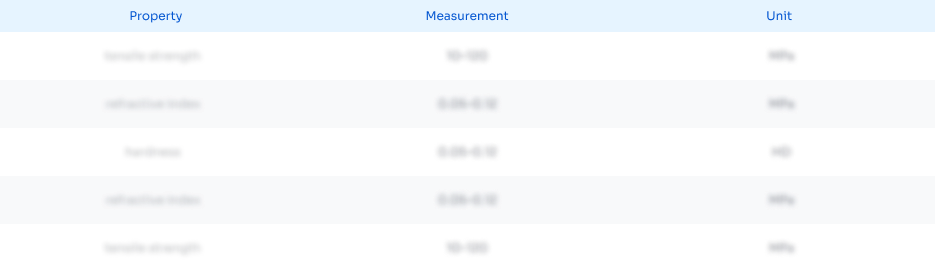
Abstract
Description
Claims
Application Information
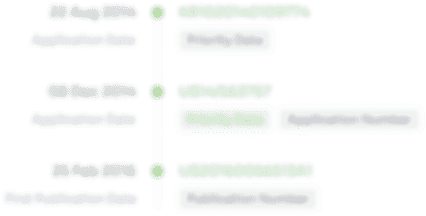
- Generate Ideas
- Intellectual Property
- Life Sciences
- Materials
- Tech Scout
- Unparalleled Data Quality
- Higher Quality Content
- 60% Fewer Hallucinations
Browse by: Latest US Patents, China's latest patents, Technical Efficacy Thesaurus, Application Domain, Technology Topic, Popular Technical Reports.
© 2025 PatSnap. All rights reserved.Legal|Privacy policy|Modern Slavery Act Transparency Statement|Sitemap|About US| Contact US: help@patsnap.com