Short-term power load prediction method based on SFO-TSVR
A short-term power load and forecasting method technology, applied in forecasting, nuclear methods, instruments, etc., can solve problems such as high data scale and hardware requirements, low precision, and slow running speed, so as to speed up convergence, ensure optimization accuracy, Effect of High Prediction Accuracy
- Summary
- Abstract
- Description
- Claims
- Application Information
AI Technical Summary
Problems solved by technology
Method used
Image
Examples
Embodiment
[0070] Such as figure 1 As shown, a short-term power load forecasting method based on SFO-TSVR includes the following steps:
[0071] S1. Obtain historical load data, meteorological data and corresponding date type data as original sample data, specifically normalize historical load data and meteorological data, and quantify the corresponding date type data to [0,1 ] range, so as to obtain the original sample data;
[0072] S2. Divide the original sample data into a training set and a test set. The first 87.5% of the data in the sample data set can be used as a training set, and the last 12.5% of the data can be used as a test set;
[0073] S3. Set the input data sequence and construct the TSVR model, specifically:
[0074] S31. Set the input data sequence specifically as follows: the meteorological data, load data and date type of the two days before the day to be predicted, the weather data, load data and date type of the day before the day to be predicted, and the weath...
PUM
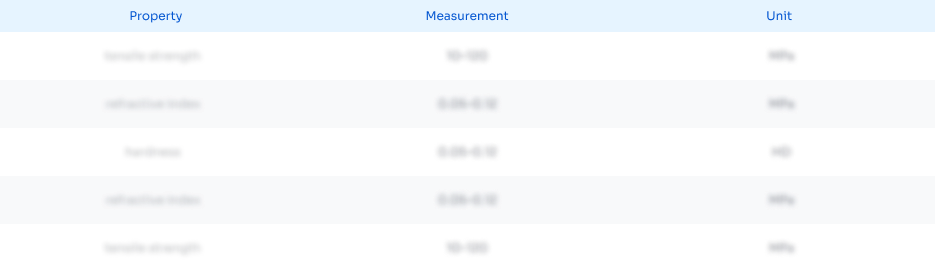
Abstract
Description
Claims
Application Information
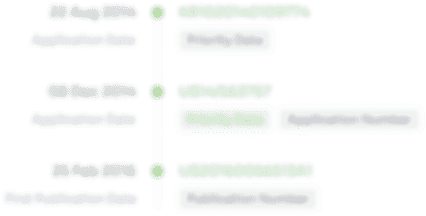
- R&D Engineer
- R&D Manager
- IP Professional
- Industry Leading Data Capabilities
- Powerful AI technology
- Patent DNA Extraction
Browse by: Latest US Patents, China's latest patents, Technical Efficacy Thesaurus, Application Domain, Technology Topic, Popular Technical Reports.
© 2024 PatSnap. All rights reserved.Legal|Privacy policy|Modern Slavery Act Transparency Statement|Sitemap|About US| Contact US: help@patsnap.com