Adaptive optimal AGC control method based on integral reinforcement learning
A technology of reinforcement learning and control methods, applied in the direction of reducing/preventing power oscillation, AC network circuits, electrical components, etc., can solve problems such as limited number of connection lines, inability to converge to the optimum, frequency deviation, etc.
- Summary
- Abstract
- Description
- Claims
- Application Information
AI Technical Summary
Problems solved by technology
Method used
Image
Examples
Embodiment 1
[0081] see Figure 1 to Figure 3 , an adaptive optimal AGC control method based on integral reinforcement learning, including the following steps:
[0082] 1) Establish a single-area power system frequency response model, and calculate the power system state space matrix;
[0083] The components of the power system include governors, turbines, generator rotors and loads.
[0084] The frequency response model of a single-area power system is described as follows:
[0085]
[0086] In the formula, ΔX g (t) is the change increment of the governor valve opening; Increment ΔX g Differential of (t); ΔP g (t) is the output variation of the generator; Increment ΔP g (t) differential; Δf(t) is the frequency error increment; is the differential of increment Δf(t); ΔI(t) is the integral increment of frequency error; is the differential of increment ΔI(t); ΔP d (t) is the load increment; T g , T t , T p are the time constants of governor, turbine and generator respecti...
Embodiment 2
[0150] An adaptive optimal AGC control method based on integral reinforcement learning, comprising the following steps:
[0151] 1) Establish a power system frequency response model
[0152] The invention mainly studies the frequency control of a single-area power system, in which typical devices include governors, turbines, generator rotors and loads, and their dynamic models can be approximated as first-order processes. The system state variable selects the change increment of the valve opening of the governor ΔX g (t), generator output variation ΔP g (t), frequency error increment Δf(t) and frequency error integral increment ΔI(t), the disturbance variable is load increment ΔP d (t), the differential equation of the system is summarized as follows:
[0153]
[0154] The system state space model is expressed as:
[0155]
[0156]
[0157]
[0158] 2) Strategy iteration of integral reinforcement learning
[0159] In the optimal control problem, a cost functio...
Embodiment 3
[0206] see Figure 4 with Figure 5 , an adaptive optimal AGC control method based on integral reinforcement learning, including the following steps:
[0207] 1) System parameter setting
[0208] The control object is figure 1 For the single zone power system shown, the governor time constant T g =0.08, turbine time constant T t =0.1, generator time constant T p =20, generator gain K p =120, governor speed drop rate R d =2.5, integral control gain K e =1.
[0209] Define the optimal control cost function as formula (6), where the state variable weight Q=I of the utility equation U(x,u), the control variable weight R=0.5, and the activation function χ(x) in the evaluation network is selected to contain 10 vector of quadratic elements The system state variable is initialized as x(0)=[0 0 0 0] T , the initial value of the evaluation network weight is The adaptive gain matrix Γ=10I, the adaptive forgetting factor β=1.2, and the sampling period of the integrated enh...
PUM
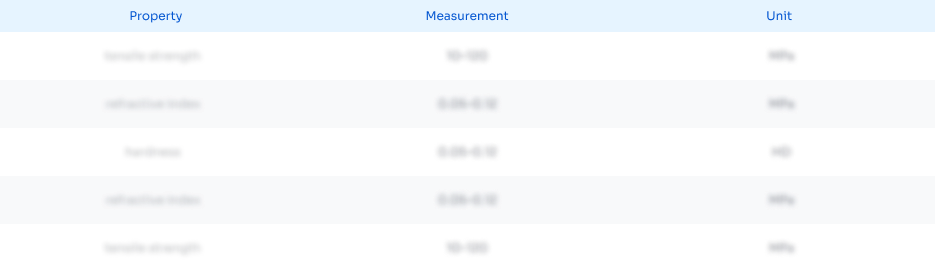
Abstract
Description
Claims
Application Information
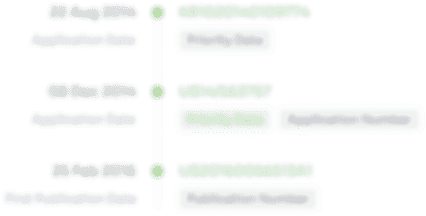
- R&D Engineer
- R&D Manager
- IP Professional
- Industry Leading Data Capabilities
- Powerful AI technology
- Patent DNA Extraction
Browse by: Latest US Patents, China's latest patents, Technical Efficacy Thesaurus, Application Domain, Technology Topic, Popular Technical Reports.
© 2024 PatSnap. All rights reserved.Legal|Privacy policy|Modern Slavery Act Transparency Statement|Sitemap|About US| Contact US: help@patsnap.com