Residual unit, network, target identification method, system and device and medium
A residual and network technology, applied in the field of residual unit, network and target recognition, can solve the problems of fixed spectrum resolution, loss of resolution information, loss of original signal waveform fine structure information, etc., and improve the accuracy of target recognition , the effect of retaining data characteristics
- Summary
- Abstract
- Description
- Claims
- Application Information
AI Technical Summary
Problems solved by technology
Method used
Image
Examples
Embodiment 1
[0070] Embodiment 1 of the present invention provides a multi-scale residual unit for processing raw underwater acoustic data. The structure of the multi-scale residual unit is as follows figure 2 As shown, it includes a residual network architecture composed of a batch normalization layer, an activation layer, and a convolutional layer for processing one-dimensional original audio waveforms. In the residual network architecture, there is at least one convolutional intermediate layer, The convolutional middle layer is formed by multiple convolutional layers in parallel, and at least one soft threshold calculation unit is provided between the convolutional middle layer and the end of the residual network architecture for soft threshold calculation and filtering.
[0071] Wherein, in practical applications, the number of convolutional intermediate layers and the number of soft threshold calculation units can be flexibly adjusted according to actual needs, which are not specifica...
Embodiment 2
[0092] Based on the multi-scale residual unit in Embodiment 1, the present invention establishes a deep neural network for processing underwater acoustic raw data, the deep neural network includes a network front end, a network middle end and a network end, and the output of the network front end The data is input to the middle end of the network, and the output data of the middle end of the network is input to the end of the network, and the middle end of the network includes at least one multi-scale residual unit described in Embodiment 1; when the middle end of the network includes multiple When there are one multi-scale residual unit, multiple multi-scale residual units are connected in sequence.
[0093] Wherein, in the embodiment of the present invention, the network front end is used to sequentially process the input data of the deep neural network through convolution processing, batch normalization processing, activation processing and pooling processing to obtain outpu...
Embodiment 3
[0117] Embodiment 3 of the present invention provides a training method for a deep neural network, where the deep neural network is the described deep neural network, and the training method includes:
[0118] Constructing the deep neural network to obtain the first deep neural network;
[0119] labeling the target radiated sound signal in the first audio data, obtaining first labeling data, and obtaining a training set based on the first labeling data;
[0120] Using the training set to train the first deep neural network to obtain a second neural network.
[0121] Wherein, in the third embodiment of the present invention, the training method also includes:
[0122] obtaining a test set based on the first labeled data;
[0123] The second deep neural network is tested using the test set.
PUM
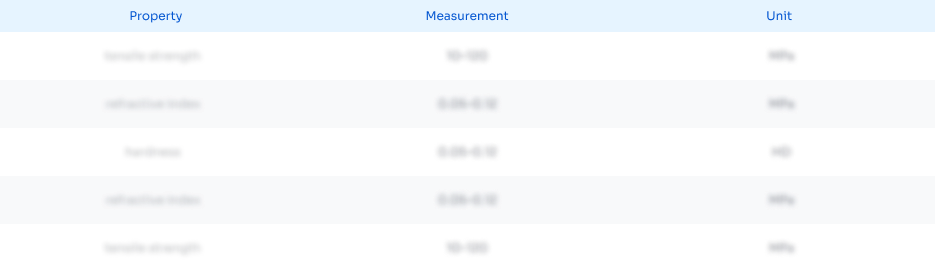
Abstract
Description
Claims
Application Information
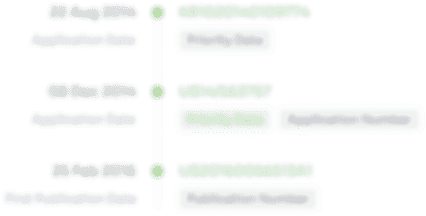
- R&D Engineer
- R&D Manager
- IP Professional
- Industry Leading Data Capabilities
- Powerful AI technology
- Patent DNA Extraction
Browse by: Latest US Patents, China's latest patents, Technical Efficacy Thesaurus, Application Domain, Technology Topic, Popular Technical Reports.
© 2024 PatSnap. All rights reserved.Legal|Privacy policy|Modern Slavery Act Transparency Statement|Sitemap|About US| Contact US: help@patsnap.com