Seismic data texture feature reconstruction method based on deep learning
A technology of seismic data and texture features, applied in neural learning methods, image data processing, biological neural network models, etc., can solve the problems of image texture damage and cannot be restored, achieve a small number of layers, restore texture feature information, and improve peak value Effects of Signal-to-Noise Ratio and Structural Similarity
- Summary
- Abstract
- Description
- Claims
- Application Information
AI Technical Summary
Problems solved by technology
Method used
Image
Examples
Embodiment 1
[0086] Step 1, in this embodiment, the size is 128 × 128; the low-resolution seismic image missing traces is randomly missing 30% traces, that is, images with a random sampling rate of 70% (such as image 3 shown). The original seismic image HR (such as Figure 5 shown) and the reference image Ref (such as Figure 4 shown).
[0087] Step 2. In order to facilitate the training of the seismic data reconstruction network based on texture migration (referred to as the seismic data reconstruction network), the low-resolution missing channel seismic image is first down-sampled (in this embodiment, it is down-sampled by 4 times), and the low-resolution image is generated. LR, and then perform the same multiple (4 times) upsampling of the low-resolution image LR to make it an upsampled low-resolution image LR_sr with the same size as the reference image Ref (this embodiment is a double-cubic upsampled low-resolution image);
[0088] Considering that the resolutions of the low-reso...
Embodiment 2
[0095] Step 1, in this embodiment, the size is 128×128; the low-resolution missing channel seismic images are images with random sampling rates of 50%, 60%, 80% and 90%.
[0096] Same as Steps 1-7 of Example 1.
[0097] Step 8. The test set is input into the trained seismic data reconstruction network. After texture extraction and texture migration, the high-resolution reconstruction image SR is reconstructed, and the peak signal-to-noise ratio (PSNR) and structural similarity (SSIM) are used as evaluation Indicators are evaluated.
[0098] Depend on Figure 9 It can be seen that as the sampling rate increases, the reconstruction results are better. The image with higher sampling rate can find more similar texture feature information when calculating the similarity with the texture feature information of the reference image, but when the sampling rate is too large, the reconstruction result is less improved.
[0099] What is not mentioned in the present invention is applica...
PUM
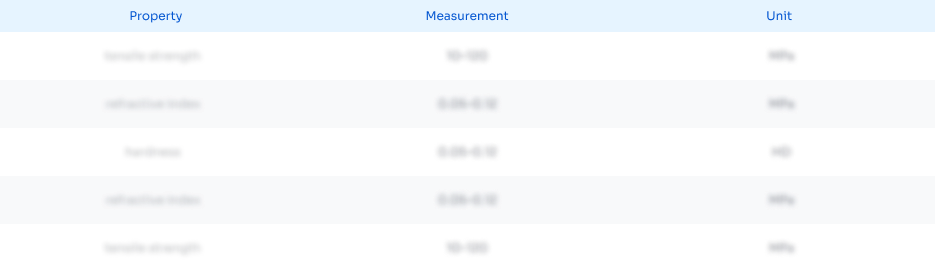
Abstract
Description
Claims
Application Information
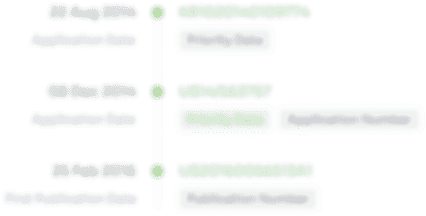
- Generate Ideas
- Intellectual Property
- Life Sciences
- Materials
- Tech Scout
- Unparalleled Data Quality
- Higher Quality Content
- 60% Fewer Hallucinations
Browse by: Latest US Patents, China's latest patents, Technical Efficacy Thesaurus, Application Domain, Technology Topic, Popular Technical Reports.
© 2025 PatSnap. All rights reserved.Legal|Privacy policy|Modern Slavery Act Transparency Statement|Sitemap|About US| Contact US: help@patsnap.com