A detection method of urine formed components based on deep learning and context-sensitive
A component detection and deep learning technology, applied in neural learning methods, image analysis, image enhancement and other directions, can solve the problems of re-inspection, easy change, missed detection, etc., to achieve accurate positioning and tracking, fast speed, and reduce labor costs. Effect
- Summary
- Abstract
- Description
- Claims
- Application Information
AI Technical Summary
Problems solved by technology
Method used
Image
Examples
Embodiment 1
[0104] like figure 1 As shown, a deep learning-based, context-sensitive urine formed component detection method includes the following steps:
[0105] S1. Establish a data set of formed components: collect video of the urine sample for labeling under the field of view of the microscopic imaging equipment, and obtain the urine video for labeling; extract images from the urine video for labeling, and obtain a time series n single-frame image data; mark the position and category of the formed elements on the single-frame image data, obtain the formed element data set, and divide the formed element data set into a training set and a test set;
[0106] S2. Establish a detection and classification model for urine formed components: build a detection and classification model for urine formed components with the functions of detection, tracking, prediction, contextual correlation matching, positioning and comparison recognition, and use training sets and test sets for model training;...
Embodiment 2
[0109] like figure 1 , 2 , 3, a deep learning-based, context-sensitive urine formed component detection method, comprising the following steps:
[0110] S1. Establish a data set of formed components: collect video of the urine sample for labeling under the field of view of the microscopic imaging equipment, and obtain the urine video for labeling; extract images from the urine video for labeling, and obtain a time series n single-frame image data; mark the position and category of the formed elements on the single-frame image data, obtain the formed element data set, and divide the formed element data set into a training set and a test set;
[0111] S2. Establish a detection and classification model for urine formed components: build a detection and classification model for urine formed components with the functions of detection, tracking, prediction, contextual correlation matching, positioning and comparison recognition, and use training sets and test sets for model traini...
Embodiment 3
[0146] like Figure 2-3 As shown, a deep learning-based, context-sensitive urine formed component detection method includes the following steps:
[0147] 1. Real-time collection of urine dynamic video under the microscope
[0148] First, each field of view is photographed and collected under the microscope imaging device to generate a time series of single field of view images.
[0149] 2. Urine Formed Component Data Labeling
[0150] Professional doctors use specific labeling tools to mark the position and category information of the formed components on the form. After the amount of labeling reaches a certain scale, the labeled data is divided into a training set and a test set according to a certain proportion to prepare data for building a neural network model.
[0151] 3. Transformer-based urine formed component detection, tracking, and recognition model construction
[0152] Construct a context-sensitive urine formed component detection, tracking, and identification a...
PUM
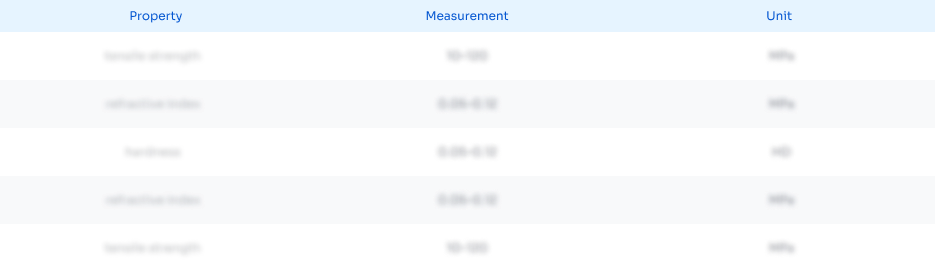
Abstract
Description
Claims
Application Information
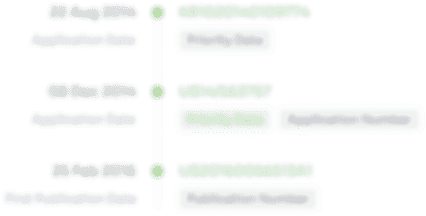
- R&D Engineer
- R&D Manager
- IP Professional
- Industry Leading Data Capabilities
- Powerful AI technology
- Patent DNA Extraction
Browse by: Latest US Patents, China's latest patents, Technical Efficacy Thesaurus, Application Domain, Technology Topic, Popular Technical Reports.
© 2024 PatSnap. All rights reserved.Legal|Privacy policy|Modern Slavery Act Transparency Statement|Sitemap|About US| Contact US: help@patsnap.com